AI and Machine Learning in Clinical Research
Artificial Intelligence (AI) and Machine Learning (ML) are revolutionizing numerous industries, and clinical research is no exception. AI refers to the simulation of human intelligence in machines programmed to think and learn like humans. Machine Learning, a subset of AI, involves the use of algorithms and statistical models to enable systems to improve their performance on tasks through experience. Together, these technologies are driving significant advancements in data analysis, predictive modeling, and decision-making processes in clinical research.
Clinical research is the cornerstone of medical advancements, aiming to discover new treatments, validate therapeutic methods, and ensure the safety and efficacy of medical interventions. Traditionally, this research relies on rigorous methodologies, extensive data collection, and meticulous analysis, often requiring substantial time and resources. Despite its critical importance, the field faces numerous challenges, including high costs, lengthy trial durations, and difficulties in patient recruitment and retention.
This blog aims to explore how AI and Machine Learning are transforming clinical research. From enhancing predictive analytics to streamlining patient recruitment and optimizing trial monitoring, these technologies are poised to address many of the industry's pain points. By examining specific applications and benefits, we will highlight the potential of AI and ML to revolutionize clinical trials, improve outcomes, and accelerate the development of new treatments.
Predictive Analytics in Clinical Research
Predictive analytics is a branch of advanced analytics that uses current and historical data, along with statistical algorithms and machine learning techniques, to forecast future events or outcomes. In the context of clinical research, predictive analytics plays a crucial role in anticipating patient responses to treatments, identifying potential side effects, and optimizing trial designs to ensure maximum efficacy and safety.
Notable Labs is at the forefront of integrating artificial intelligence (AI) into medical research, particularly in predictive precision medicine. Their AI-driven platform has shown remarkable success in drug development, exemplified by its ability to predict patient responses to treatments like venetoclax combined with decitabine for Acute Myeloid Leukemia (AML). By leveraging expansive datasets from patient samples, Notable Labs enhances the precision of drug efficacy predictions, thereby improving patient outcomes, reducing development risks, and increasing cost-efficiency in drug development
How AI Algorithms Predict Patient Outcomes and Treatment Responses
AI algorithms excel at processing vast amounts of data from diverse sources, such as electronic health records, genetic profiles, and clinical trial results. These algorithms identify patterns and correlations that may not be immediately apparent to human researchers. For instance, machine learning models can analyze data to predict which patients are most likely to respond positively to a new drug based on their genetic makeup and medical history. Similarly, AI can forecast potential adverse reactions, enabling researchers to adjust treatment plans proactively.
One notable example of AI in predictive analytics is IBM Watson Health's use of machine learning to predict the outcomes of cancer treatments. By analyzing millions of data points from cancer patients worldwide, Watson Health can recommend personalized treatment plans, predict treatment success rates, and identify patients at high risk for complications.
Another successful implementation is the use of AI by the company Tempus, which specializes in precision medicine. Tempus uses machine learning algorithms to analyze clinical and molecular data, helping oncologists develop individualized treatment plans for cancer patients. Their platform integrates data from clinical trials, electronic health records, and genomic sequencing to provide comprehensive insights into patient care.
Benefits: Improved Accuracy, Personalized Treatment Plans, Reduced Costs
The integration of predictive analytics in clinical research offers several significant benefits:
- Improved Accuracy: AI algorithms can process and analyze data with greater accuracy and speed than traditional methods. This leads to more precise predictions regarding patient outcomes and treatment efficacy, reducing the likelihood of errors and increasing the reliability of clinical trial results.
- Personalized Treatment Plans: By leveraging predictive analytics, researchers can develop tailored treatment plans that consider an individual's unique genetic makeup, lifestyle, and medical history. Personalized medicine increases the chances of treatment success and minimizes the risk of adverse effects.
- Reduced Costs: The ability to predict patient responses and optimize trial designs can significantly reduce the time and resources required for clinical trials. Efficient patient recruitment, targeted treatment plans, and early identification of potential issues contribute to cost savings and faster drug development processes.
Predictive analytics, powered by AI and machine learning, is transforming clinical research by enhancing the accuracy and efficiency of trials, enabling personalized medicine, and reducing overall costs. As these technologies continue to advance, their impact on the field will only grow, paving the way for more effective and patient-centered healthcare solutions.
Enhancing Patient Recruitment
Challenges in Traditional Patient Recruitment
Patient recruitment is one of the most critical and challenging aspects of clinical research. Traditional recruitment methods often face several hurdles, including:
- Limited Reach: Conventional recruitment strategies rely heavily on physicians' networks and existing patient databases, which may not reach a broad or diverse patient population.
- Time-Consuming: The process of identifying, screening, and enrolling eligible patients is often lengthy and labor-intensive, delaying trial commencement.
- High Costs: Recruiting patients can be costly due to extensive advertising, outreach programs, and administrative efforts.
- Low Participation Rates: Many eligible patients may be unaware of clinical trials or hesitant to participate due to a lack of information or trust in the process.
AI-Driven Solutions for Identifying and Enrolling Suitable Candidates
AI and machine learning offer innovative solutions to address these challenges and enhance patient recruitment. By leveraging vast amounts of data and advanced algorithms, AI can streamline the recruitment process in several ways:
- Data Mining and Analysis: AI can sift through electronic health records, social media, and other data sources to identify potential candidates who meet the trial's inclusion criteria.
- Predictive Modeling: Machine learning models can predict which patients are most likely to participate in a trial based on historical data, patient demographics, and behavioral patterns.
- Automated Outreach: AI-powered platforms can automate personalized communication with potential participants, providing them with relevant information and addressing their concerns promptly.
- Optimized Matching: AI can match patients to trials with greater precision, considering multiple factors such as genetic markers, medical history, and current health status.
Examples of AI Tools/Platforms Used in Patient Recruitment
Several AI-driven tools and platforms are transforming patient recruitment in clinical research:
- Deep 6 AI: This platform uses natural language processing and machine learning to analyze clinical data and identify eligible patients for trials in real-time. It helps researchers quickly find and enroll suitable candidates from large patient databases.
- Antidote: Antidote uses AI to connect patients with clinical trials by analyzing patient data and trial criteria. The platform provides a user-friendly interface for patients to find and apply for trials that match their conditions.
- TriNetX: TriNetX leverages real-world data and AI to identify patient cohorts for clinical trials. It enables researchers to perform feasibility studies and recruit participants more efficiently by accessing a global network of healthcare organizations.
Impact on Diversity and Inclusion in Clinical Trials
One of the significant benefits of AI-enhanced patient recruitment is its potential to improve diversity and inclusion in clinical trials. Traditionally, clinical trials have struggled with underrepresentation of certain demographic groups, leading to biased results and limited generalizability of findings. AI can address this issue by:
- Broadening Outreach: AI can identify and reach underrepresented populations through diverse data sources, ensuring a more inclusive recruitment process.
- Reducing Bias: Machine learning algorithms can be trained to recognize and mitigate biases in patient selection, promoting fair and equitable participation.
- Personalized Engagement: AI-driven platforms can tailor communication strategies to resonate with diverse populations, addressing specific cultural, linguistic, and informational needs.
By enhancing patient recruitment through AI and machine learning, clinical research can become more efficient, inclusive, and effective. These technologies not only expedite the recruitment process but also ensure that trial populations better reflect the diversity of the real world, ultimately leading to more robust and generalizable study outcomes.
Optimizing Trial Design and Planning
Role of AI in Designing Efficient and Effective Clinical Trials
AI is revolutionizing the design and planning phases of clinical trials by introducing advanced tools and methodologies that enhance efficiency and effectiveness. Traditional trial design often relies on historical data and expert intuition, which can be limited in scope and accuracy. AI, on the other hand, utilizes vast datasets and sophisticated algorithms to create more precise and adaptive trial designs.
AI-driven trial design involves:
- Data-Driven Protocol Development: AI can analyze extensive datasets to identify optimal trial protocols, including dosing regimens, patient stratification criteria, and endpoint definitions. This leads to more scientifically sound and tailored trial designs.
- Simulation and Modeling: AI can simulate various trial scenarios to predict outcomes and identify potential challenges before the trial begins. This helps in refining the design to avoid common pitfalls and ensure robustness.
- Automating Administrative Tasks: AI can streamline administrative processes, such as regulatory document preparation and submission, freeing up researchers to focus on core scientific activities.
Adaptive Trial Designs and Real-Time Modifications
Adaptive trial designs allow for modifications to be made to the trial protocol based on interim data analyses. AI plays a crucial role in facilitating these adaptive designs by enabling real-time data processing and decision-making.
- Interim Data Analysis: AI can continuously monitor and analyze data as it is collected during the trial. This enables researchers to make informed decisions about modifications, such as adjusting dosages, changing patient cohorts, or even halting the trial if necessary.
- Flexibility and Responsiveness: Adaptive designs, supported by AI, allow trials to be more flexible and responsive to emerging data. This can lead to more efficient use of resources and faster identification of effective treatments.
- Risk Mitigation: Real-time modifications can help mitigate risks associated with unforeseen adverse events or lack of efficacy, ensuring patient safety and optimizing trial outcomes.
Predictive Models for Determining Trial Feasibility and Success Rates
AI-powered predictive models are invaluable for assessing the feasibility and potential success of clinical trials before they commence. These models leverage historical data and machine learning techniques to forecast various aspects of the trial.
- Feasibility Assessment: Predictive models can evaluate the likelihood of recruiting sufficient patients within a given timeframe, based on factors such as disease prevalence, patient demographics, and geographic distribution. This helps in planning realistic and achievable trial timelines.
- Success Rate Prediction: AI can analyze past trial data to predict the probability of achieving the desired outcomes. This includes evaluating the efficacy and safety profiles of the investigational treatments. Accurate predictions can inform go/no-go decisions, thereby optimizing resource allocation.
- Operational Efficiency: By predicting potential challenges and success rates, AI helps in optimizing resource allocation and operational planning. This includes staffing needs, site selection, and budget planning, ensuring that the trial is conducted efficiently and cost-effectively.
Real-Time Monitoring and Data Management
AI-Powered Tools for Real-Time Monitoring of Clinical Trials
The real-time monitoring of clinical trials is critical for ensuring patient safety, data integrity, and overall trial success. AI-powered tools have transformed this aspect of clinical research by providing continuous oversight and rapid response capabilities. These tools use advanced algorithms to process data as it is collected, offering immediate insights and identifying potential issues before they escalate.
- Continuous Data Collection and Analysis: AI tools can continuously gather and analyze data from various sources, including electronic health records, wearable devices, and patient-reported outcomes. This real-time analysis helps in identifying trends, anomalies, and critical events as they occur.
- Automated Alerts and Notifications: AI systems can automatically generate alerts and notifications for researchers and trial managers when predefined thresholds or unusual patterns are detected. This ensures timely intervention and corrective actions.
- Patient Monitoring: AI-powered wearables and remote monitoring devices can track vital signs and other health parameters of trial participants in real-time. This continuous monitoring enhances patient safety and adherence to the trial protocol.
Ensuring Data Integrity and Compliance Through Machine Learning Algorithms
Maintaining data integrity and regulatory compliance is paramount in clinical research. Machine learning algorithms play a significant role in ensuring that data collected during trials is accurate, complete, and adheres to regulatory standards.
- Data Validation and Cleaning: Machine learning algorithms can automatically validate and clean data, identifying and correcting errors or inconsistencies. This ensures that the data used for analysis is of high quality.
- Regulatory Compliance: AI can assist in monitoring compliance with regulatory requirements by tracking and documenting all trial activities. Machine learning models can analyze data to ensure adherence to Good Clinical Practice (GCP) guidelines and other regulatory frameworks.
- Fraud Detection: AI algorithms can detect patterns indicative of fraudulent or non-compliant behavior, such as fabricated data or protocol deviations. Early detection of such issues helps maintain the integrity of the trial.
Examples of Platforms and Technologies Used for Trial Monitoring
Several AI-powered platforms and technologies are revolutionizing the real-time monitoring and data management of clinical trials:
- Medidata Rave: Medidata Rave is a cloud-based platform that uses AI to streamline data collection, management, and analysis in clinical trials. It provides real-time insights, automates data validation, and ensures compliance with regulatory standards.
- Covance Xcellerate: Covance Xcellerate is an AI-driven platform that offers comprehensive monitoring solutions, including risk-based monitoring and real-time data analytics. It helps identify potential risks and optimize trial performance.
- PRA Health Sciences Symphony: Symphony is an integrated platform that leverages AI to enhance trial monitoring and data management. It provides real-time data visualization, predictive analytics, and automated reporting to support effective trial oversight.
- Veristat Virtual Trials: Veristat’s platform enables the conduct of decentralized and virtual trials using AI-powered remote monitoring and data collection tools. This technology ensures continuous patient monitoring and real-time data capture, improving trial efficiency and participant engagement.
Improving Data Analysis and Interpretation
AI's Role in Handling and Analyzing Large Datasets
One of the most significant challenges in clinical research is managing and analyzing the vast amounts of data generated during trials. AI excels in handling large datasets by leveraging its computational power and advanced algorithms to process and analyze data efficiently. This capability is particularly crucial in clinical research, where data volume and complexity can be overwhelming.
- Scalability: AI systems can scale to accommodate large datasets, processing millions of data points from diverse sources such as electronic health records, genomic data, and real-world evidence. This scalability ensures that no valuable data is overlooked.
- Data Integration: AI can integrate data from multiple sources, creating a comprehensive dataset that provides a holistic view of patient health and treatment outcomes. This integration is essential for drawing accurate and meaningful conclusions.
- Automated Data Processing: AI automates many data processing tasks, such as data cleaning, normalization, and transformation. This automation reduces the time and effort required for data preparation, allowing researchers to focus on analysis and interpretation.
Machine Learning Models for Identifying Patterns and Insights
Machine learning models are at the core of AI's ability to identify patterns and insights within large datasets. These models learn from historical data to make predictions, detect trends, and uncover hidden relationships that may not be evident through traditional analysis methods.
- Pattern Recognition: Machine learning algorithms can recognize complex patterns in clinical data, such as correlations between genetic markers and treatment responses or the progression of disease symptoms over time. These patterns provide valuable insights into patient outcomes and potential treatment pathways.
- Predictive Analytics: Machine learning models can predict patient outcomes based on historical data. For example, predictive models can estimate the likelihood of treatment success, identify patients at risk of adverse events, and forecast disease progression. These predictions help researchers make data-driven decisions and tailor interventions to individual patients.
- Anomaly Detection: AI algorithms can detect anomalies or outliers in the data, which may indicate potential issues such as data entry errors, protocol deviations, or unexpected patient responses. Early detection of anomalies ensures the integrity and reliability of the trial data.
Enhancing the Speed and Accuracy of Data Interpretation in Clinical Research
AI significantly enhances the speed and accuracy of data interpretation in clinical research, enabling researchers to derive meaningful insights more quickly and reliably.
- Accelerated Analysis: AI algorithms can analyze large datasets in a fraction of the time required by traditional methods. This acceleration allows researchers to rapidly generate insights and make timely decisions, ultimately speeding up the trial process.
- Increased Accuracy: AI reduces the risk of human error in data analysis by automating complex calculations and statistical analyses. The precision of machine learning models ensures that the interpretations are accurate and reproducible.
- Visualizations and Reporting: AI tools often include advanced data visualization capabilities, enabling researchers to present findings in a clear and understandable manner. Automated reporting features generate comprehensive reports that highlight key insights and trends, facilitating communication and decision-making.
For instance, Google's DeepMind has developed AI algorithms capable of analyzing medical images and identifying disease markers with remarkable accuracy. In clinical research, such technologies can expedite the analysis of imaging data, leading to quicker and more accurate diagnoses and treatment plans.
Another example is the use of AI by the company Atomwise, which applies machine learning to analyze molecular data and predict the efficacy of potential drug candidates. By rapidly screening millions of compounds, Atomwise accelerates the drug discovery process and identifies promising candidates for further investigation.
AI's role in improving data analysis and interpretation is transforming clinical research by making it more efficient, accurate, and insightful. As AI technologies continue to advance, their impact on clinical trials will grow, leading to faster development of new treatments and improved patient outcomes.
Addressing Ethical and Regulatory Considerations
Ethical Challenges in Integrating AI into Clinical Research
The integration of AI into clinical research brings about several ethical challenges that need to be carefully addressed to ensure the responsible use of these technologies.
- Bias and Fairness: AI algorithms can inadvertently perpetuate or amplify existing biases in the data, leading to unfair treatment or exclusion of certain patient groups. Ensuring that AI systems are trained on diverse and representative datasets is crucial to mitigate this risk.
- Informed Consent: The use of AI in clinical trials necessitates clear communication with participants regarding how their data will be used, processed, and protected. Obtaining informed consent in a transparent manner is essential to uphold participants' rights and autonomy.
- Privacy and Confidentiality: AI systems often require access to sensitive patient data, raising concerns about data privacy and confidentiality. Implementing robust data protection measures and ensuring compliance with privacy regulations is vital to safeguard patient information.
- Accountability: Determining accountability for decisions made by AI systems can be challenging, especially in complex clinical settings. Establishing clear guidelines and accountability frameworks is necessary to ensure that AI tools are used responsibly and ethically.
Regulatory Guidelines and Frameworks for AI in Clinical Trials
Regulatory bodies are developing guidelines and frameworks to ensure the safe and ethical use of AI in clinical trials. These regulations aim to address the unique challenges posed by AI technologies and provide a structured approach to their implementation.
- FDA and EMA Guidelines: The U.S. Food and Drug Administration (FDA) and the European Medicines Agency (EMA) have issued guidelines on the use of AI and machine learning in medical research. These guidelines emphasize the importance of transparency, validation, and ongoing monitoring of AI systems.
- Good Machine Learning Practice (GMLP): GMLP frameworks provide best practices for the development, validation, and deployment of AI systems in clinical research. These practices include rigorous testing, continuous monitoring, and regular updates to ensure AI models remain accurate and reliable.
- Ethics Committees and Institutional Review Boards (IRBs): Ethics committees and IRBs play a crucial role in overseeing the ethical aspects of AI integration in clinical trials. They review study protocols, ensure informed consent, and monitor compliance with ethical standards.
Ensuring Transparency, Accountability, and Patient Safety
Ensuring transparency, accountability, and patient safety is paramount when integrating AI into clinical research. Implementing the following measures can help achieve these goals:
- Transparency: Providing clear and comprehensive information about how AI systems work, the data they use, and their decision-making processes is essential for building trust among researchers, participants, and regulatory bodies. Transparent reporting of AI methodologies and results ensures that stakeholders can assess the reliability and validity of AI-driven insights.
- Accountability: Establishing clear lines of accountability for the development, deployment, and oversight of AI systems is critical. This includes defining the roles and responsibilities of AI developers, researchers, and clinical trial sponsors. Accountability frameworks should also address the ethical use of AI and ensure that any issues or errors are promptly addressed.
- Patient Safety: Prioritizing patient safety involves rigorous testing and validation of AI systems before they are deployed in clinical trials. Continuous monitoring and updating of AI models are necessary to ensure they remain accurate and effective over time. Implementing fail-safes and manual oversight mechanisms can help mitigate risks and address any unexpected outcomes promptly.
For example, the FDA's "Digital Health Innovation Action Plan" outlines a risk-based approach to regulating digital health technologies, including AI. This plan emphasizes the importance of maintaining high standards for safety and efficacy while fostering innovation in AI-driven healthcare solutions.
Another initiative, the AI for Health Imaging (AI4HI) consortium in Europe, focuses on developing standardized evaluation frameworks and regulatory guidelines for AI in medical imaging. This consortium aims to ensure that AI technologies used in clinical research adhere to rigorous ethical and regulatory standards.
Addressing ethical and regulatory considerations is crucial for the responsible integration of AI into clinical research. By implementing robust guidelines, ensuring transparency and accountability, and prioritizing patient safety, the clinical research community can harness the transformative potential of AI while upholding ethical standards and protecting participants' rights.
Future Prospects and Innovations
Emerging Trends and Future Directions in AI and Machine Learning in Clinical Research
The future of AI and machine learning in clinical research is brimming with potential and innovation. As these technologies continue to evolve, several emerging trends are poised to shape the landscape of clinical trials and medical research:
- Personalized Medicine: AI and machine learning are driving the shift towards personalized medicine, where treatments are tailored to individual patients based on their genetic makeup, lifestyle, and health data. This approach promises more effective and targeted therapies with fewer side effects.
- Integration with Real-World Data: The use of real-world data (RWD), such as patient records, wearable device data, and social determinants of health, is becoming increasingly common. AI can integrate and analyze RWD to provide more comprehensive insights and improve the external validity of clinical trials.
- Decentralized Clinical Trials: AI facilitates the conduct of decentralized or virtual clinical trials, where participants can enroll and participate remotely. This trend increases accessibility and convenience for patients, leading to faster recruitment and more diverse participant populations.
- Advanced Genomic Analysis: AI-driven genomic analysis is advancing rapidly, enabling researchers to identify novel biomarkers and genetic variations that influence disease and treatment responses. This capability enhances the precision and efficiency of drug development.
- Enhanced Drug Discovery: AI is revolutionizing drug discovery by predicting molecular interactions, identifying potential drug candidates, and optimizing drug design. These advancements can significantly reduce the time and cost associated with bringing new therapies to market.
Potential Breakthroughs and Innovations on the Horizon
Several breakthroughs and innovations in AI and machine learning are on the horizon, promising to further transform clinical research:
- AI-Driven Biomarker Discovery: Identifying biomarkers that predict disease progression and treatment response is crucial for personalized medicine. AI algorithms can analyze large datasets to discover new biomarkers, leading to more precise diagnostics and targeted therapies.
- Automated Trial Design: Future AI systems may be capable of fully automating the design of clinical trials, from protocol development to patient stratification. This automation could enhance trial efficiency, reduce costs, and improve trial outcomes.
- Predictive Maintenance for Trials: AI can predict potential disruptions in clinical trials, such as patient dropouts or supply chain issues, allowing researchers to proactively address these challenges and ensure trial continuity.
- Enhanced Patient Engagement: AI-powered chatbots and virtual assistants can improve patient engagement by providing real-time support, answering questions, and reminding participants of study requirements. This engagement can enhance compliance and retention rates.
- AI Ethics and Explainability: Advances in AI ethics and explainability will ensure that AI systems are transparent, fair, and accountable. These innovations will build trust among stakeholders and facilitate the broader adoption of AI in clinical research.
Long-Term Impact on the Clinical Research Landscape
The long-term impact of AI and machine learning on clinical research is profound and far-reaching. These technologies are set to redefine how clinical trials are conducted and how new therapies are developed:
- Accelerated Drug Development: AI will significantly speed up the drug development process by optimizing trial design, enhancing patient recruitment, and improving data analysis. This acceleration will bring new treatments to patients faster and more efficiently.
- Improved Patient Outcomes: By enabling personalized medicine and more precise treatments, AI will lead to better patient outcomes, reduced adverse effects, and improved quality of life for patients.
- Greater Accessibility: Decentralized trials and AI-driven recruitment strategies will make clinical trials more accessible to diverse populations, ensuring that research findings are more generalizable and inclusive.
- Cost Reduction: AI will reduce the costs associated with clinical trials by streamlining processes, automating routine tasks, and minimizing the need for physical infrastructure. These savings can be reinvested in further research and innovation.
- Ethical and Transparent Research: Advances in AI ethics and transparency will ensure that clinical research is conducted responsibly and ethically, protecting patient rights and maintaining public trust.
Recap of key points discussed
Throughout this blog, we have explored how AI and machine learning are revolutionizing various aspects of clinical research, from predictive analytics to patient recruitment and trial monitoring. Here’s a recap of the key points discussed:
- Predictive Analytics: AI algorithms enhance predictive analytics by accurately forecasting patient outcomes and treatment responses, leading to improved accuracy, personalized treatment plans, and reduced costs.
- Enhancing Patient Recruitment: AI-driven solutions address the challenges of traditional patient recruitment by efficiently identifying and enrolling suitable candidates, thus promoting diversity and inclusion in clinical trials.
- Optimizing Trial Design and Planning: AI facilitates adaptive trial designs, real-time modifications, and predictive models to determine trial feasibility and success rates, ultimately improving trial efficiency and outcomes.
- Real-Time Monitoring and Data Management: AI-powered tools provide continuous oversight and rapid response capabilities, ensuring data integrity, regulatory compliance, and patient safety through advanced monitoring and analysis.
- Improving Data Analysis and Interpretation: AI handles large datasets, identifies patterns and insights, and enhances the speed and accuracy of data interpretation, leading to more robust and reliable clinical research.
- Addressing Ethical and Regulatory Considerations: The integration of AI in clinical research requires addressing ethical challenges, adhering to regulatory guidelines, and ensuring transparency, accountability, and patient safety.
- Future Prospects and Innovations: Emerging trends and potential breakthroughs in AI and machine learning promise to further transform clinical research, leading to accelerated drug development, improved patient outcomes, greater accessibility, and cost reductions.
The Transformative Potential of AI and Machine Learning in Clinical Research
The transformative potential of AI and machine learning in clinical research is immense. These technologies are reshaping the landscape of clinical trials by enhancing efficiency, accuracy, and personalization. AI’s ability to process and analyze vast amounts of data, predict outcomes, and optimize trial designs is accelerating the development of new treatments and improving patient care. Moreover, AI-driven innovations are making clinical trials more inclusive, ethical, and accessible, ensuring that the benefits of medical research are more broadly distributed.
As AI and machine learning continue to evolve, their integration into clinical trials will become increasingly sophisticated and impactful. The future of clinical research with AI integration is promising, with the potential to revolutionize how new therapies are discovered, developed, and delivered to patients. Key aspects to consider for the future include:
- Continuous Innovation: Ongoing advancements in AI and machine learning will introduce new tools and methodologies, further enhancing the efficiency and effectiveness of clinical trials.
- Ethical Considerations: Ensuring the ethical use of AI will remain a priority, with continuous efforts to address biases, protect patient privacy, and maintain transparency and accountability.
- Collaboration and Standardization: Collaboration among researchers, regulatory bodies, and AI developers will be essential to create standardized frameworks and best practices for the ethical and effective use of AI in clinical research.
- Patient-Centric Approaches: AI-driven innovations will continue to prioritize patient-centric approaches, focusing on personalized medicine and improving patient engagement and outcomes.
In conclusion, the integration of AI and machine learning in clinical research is not just a trend but a fundamental shift towards more efficient, accurate, and inclusive medical research. As we embrace these technologies, we move closer to a future where clinical trials are faster, more reliable, and more reflective of diverse patient populations, ultimately leading to better healthcare for all.
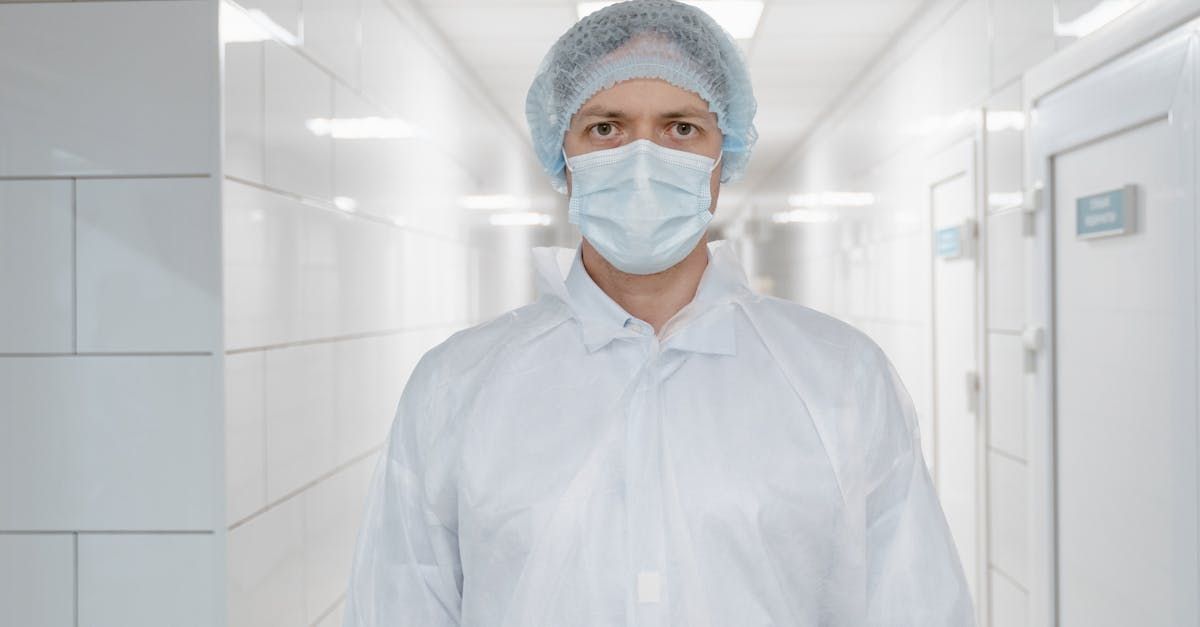
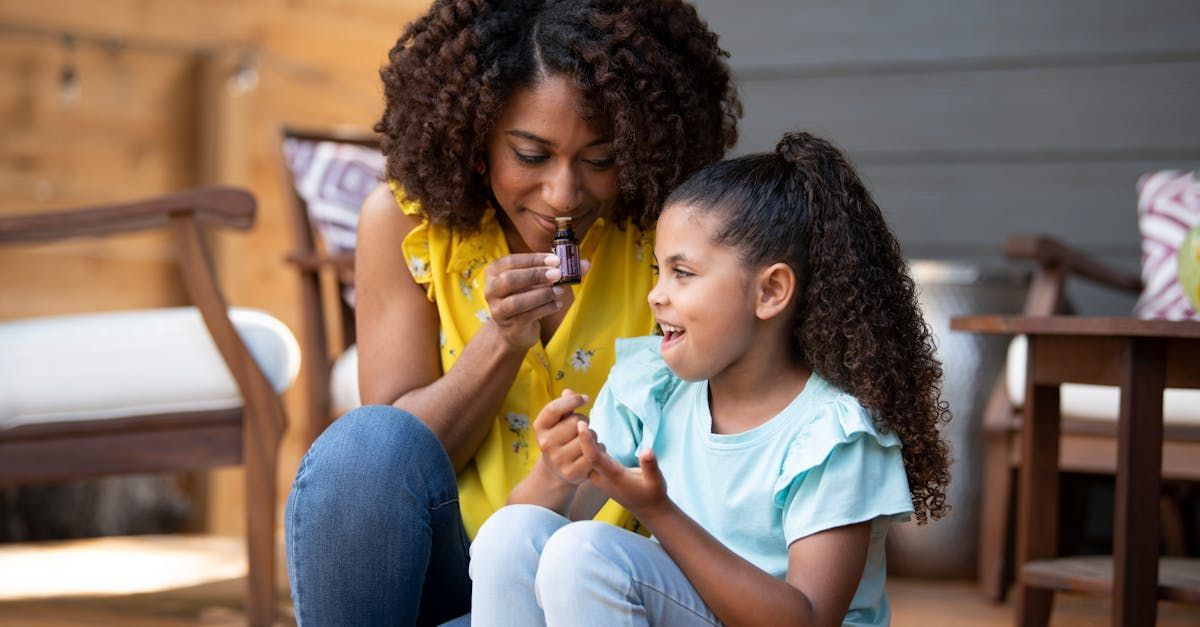
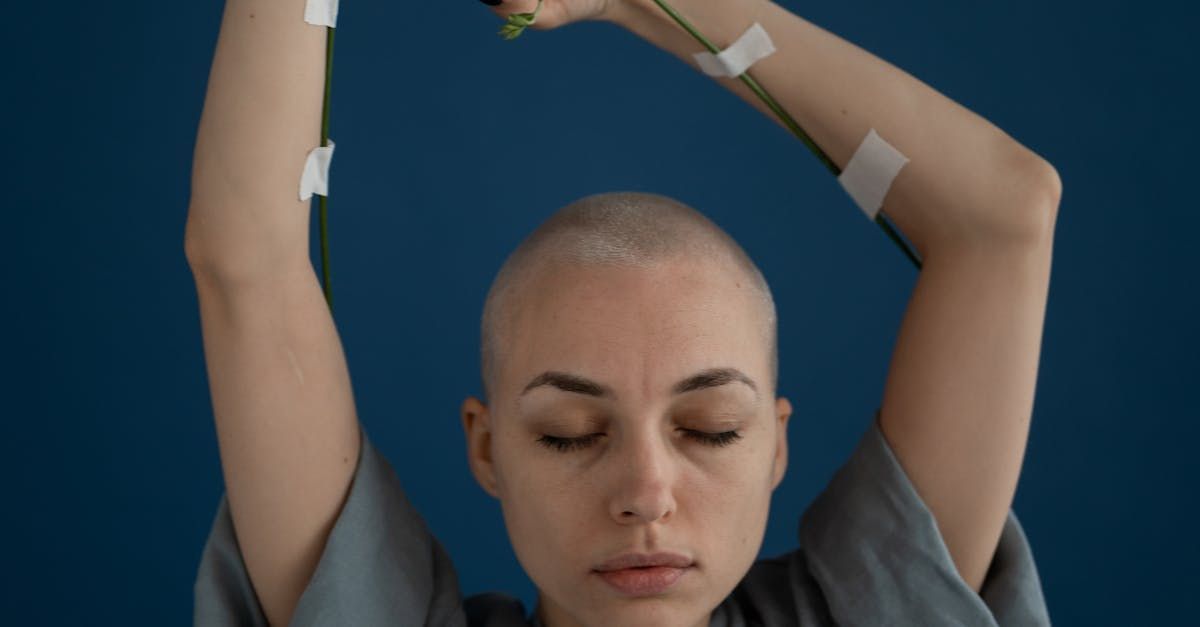
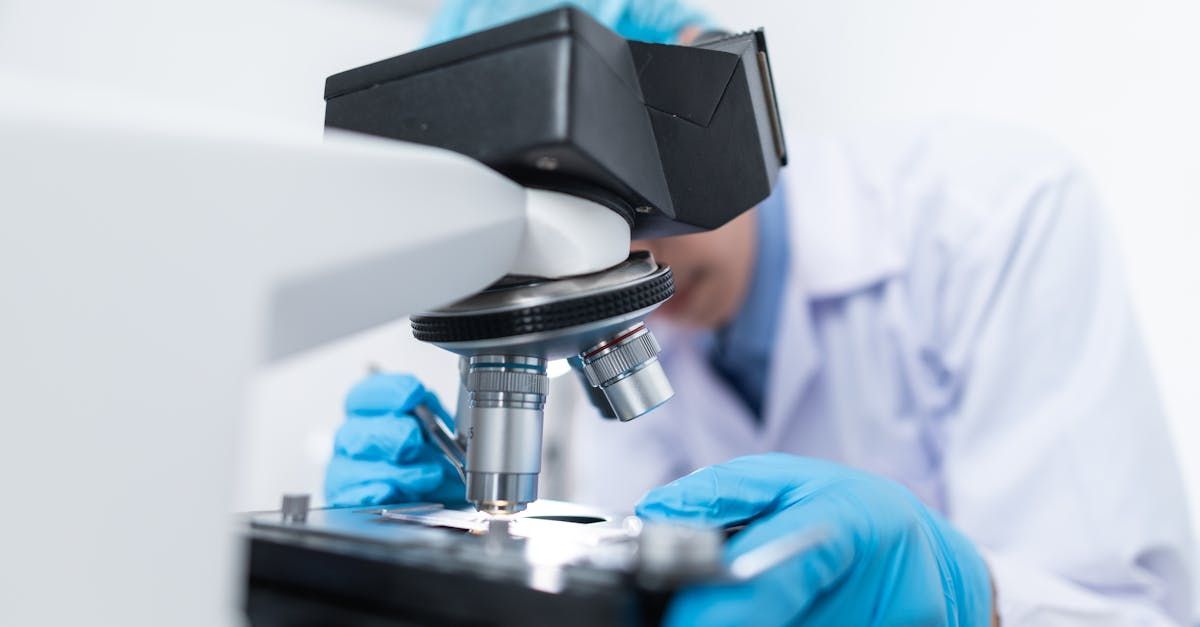
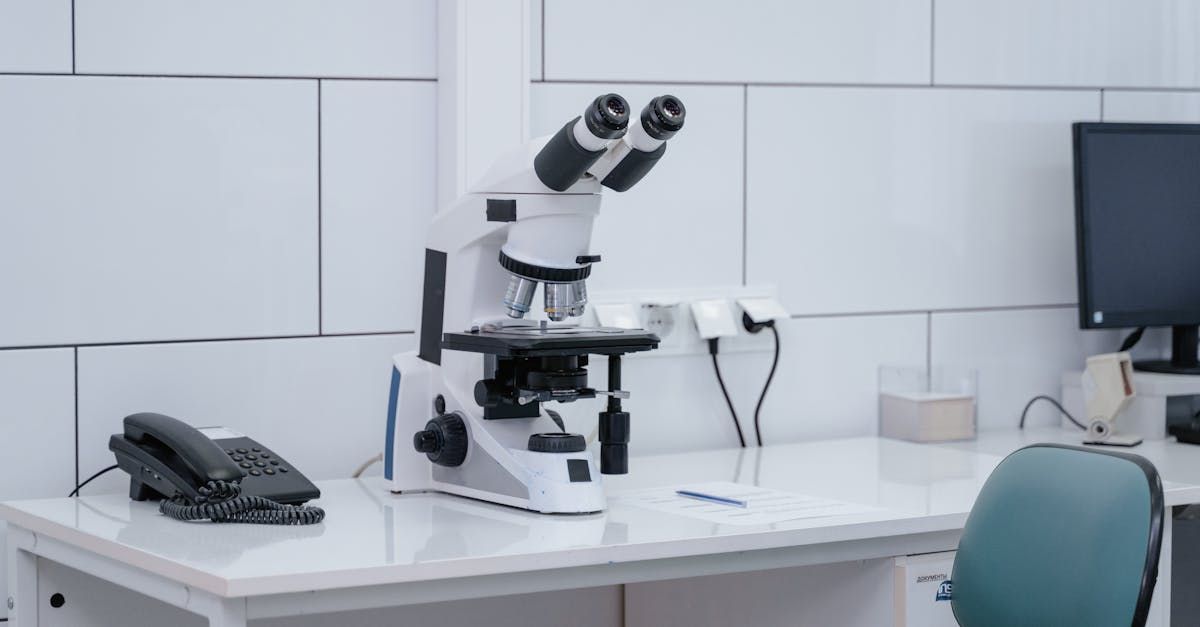
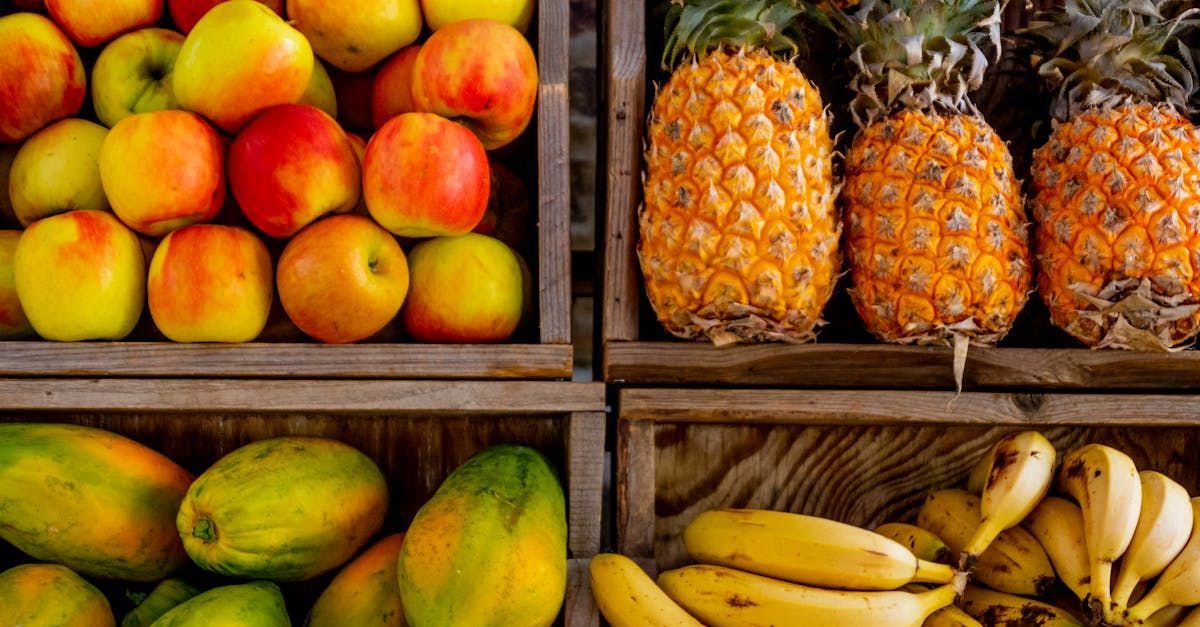
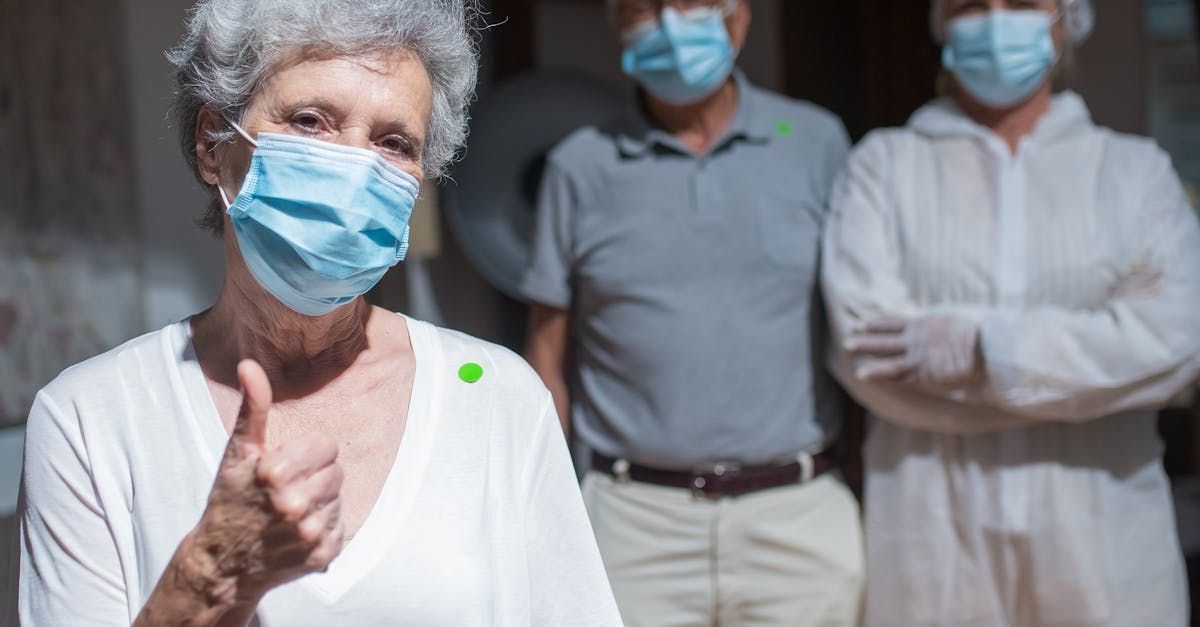
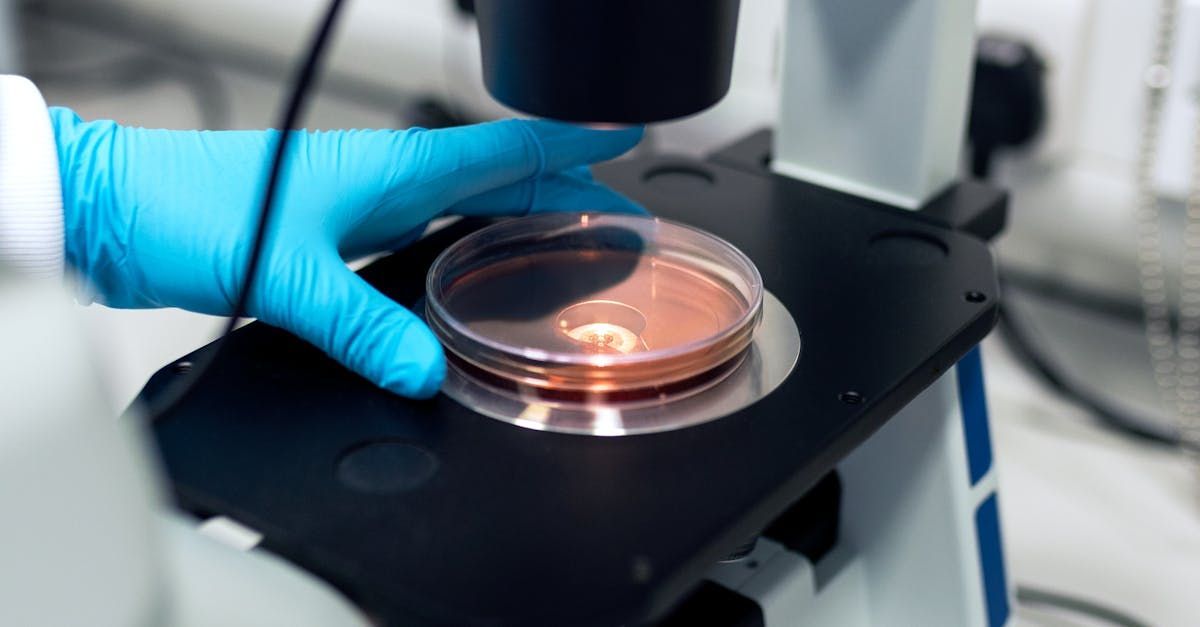
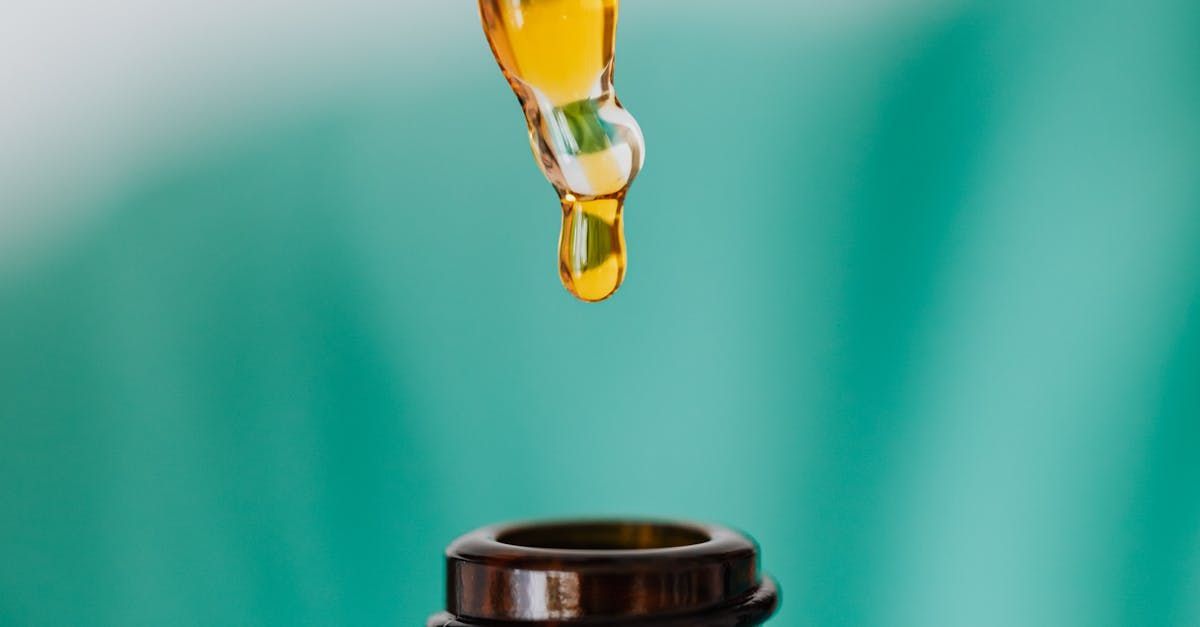
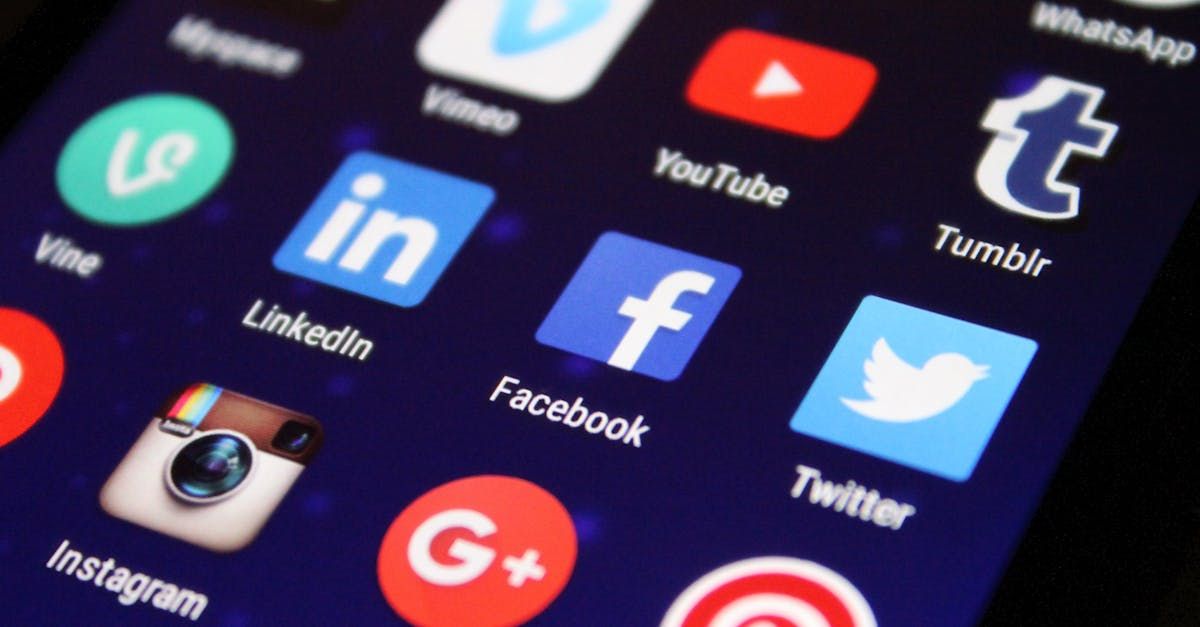