Current Trends in Clinical Research Methodologies
Clinical research is the cornerstone of medical advancement, providing the necessary foundation for developing new treatments, therapies, and understanding diseases. Through meticulously designed studies, clinical research enables the evaluation of the safety and efficacy of new interventions before they reach the public. This process is vital for ensuring that new medical products can deliver benefits while minimizing risks to patients.
Over the years, the landscape of clinical research has been continuously evolving. Traditional methodologies, while effective, often involve lengthy timelines, high costs, and complex logistics. However, recent advancements in technology and innovative approaches are transforming the way clinical trials are conducted. These new methodologies aim to enhance the efficiency and accuracy of trials, ultimately accelerating the pace at which new treatments become available to patients.
In this blog, we will explore the latest trends in clinical research methodologies. From adaptive trials to decentralized approaches and the integration of real-world evidence, we will highlight how these innovations are reshaping the clinical trial landscape. Our goal is to provide insights into how these cutting-edge methods are improving the quality and speed of clinical research, benefiting both researchers and patients alike.
Adaptive Clinical Trials
Definition and Explanation of Adaptive Clinical Trials
Adaptive clinical trials represent a significant shift from traditional fixed-design trials. In a conventional trial, the design is set in stone before the study begins, with no changes allowed once patient enrollment starts. In contrast, adaptive trials use interim data analysis to make real-time modifications to the study. These modifications can include changes to the sample size, dosage, patient selection criteria, or even the endpoints of the study. This dynamic approach allows researchers to respond to emerging data, potentially making trials more efficient and effective.
Benefits: Flexibility, Efficiency, Cost-Effectiveness
- Flexibility: Adaptive trials offer the flexibility to make adjustments based on interim results, reducing the likelihood of trial failure and enabling more precise targeting of patient populations. This approach helps in identifying the most promising treatments earlier in the process.
- Efficiency: By incorporating real-time data analysis, adaptive trials can often reach conclusions faster than traditional trials. This increased efficiency can lead to earlier identification of effective treatments or the termination of ineffective ones, saving valuable time and resources.
- Cost-Effectiveness: Adaptive trials can be more cost-effective by requiring fewer patients or shorter durations to achieve conclusive results. Adjustments made during the trial can help avoid unnecessary expenses associated with ineffective treatment arms or prolonged study periods.
Case Studies/Examples of Successful Adaptive Trials
- The I-SPY 2 Trial: One of the most well-known adaptive trials is the I-SPY 2 trial, which focused on breast cancer treatments. This trial utilized an adaptive design to evaluate multiple therapies simultaneously. Treatments showing early promise were quickly advanced to later stages, while less effective ones were discontinued. This approach significantly accelerated the identification of effective therapies for specific patient subgroups, making the trial a model for future oncology studies.
- The REMAP-CAP Trial: During the COVID-19 pandemic, the REMAP-CAP trial used an adaptive platform to evaluate multiple treatments for critically ill patients with community-acquired pneumonia, including those with COVID-19. The adaptive design allowed the trial to rapidly incorporate new treatments and adapt based on real-time results, providing timely and valuable insights into the most effective therapies for managing severe respiratory infections.
- The BATTLE Trial: The BATTLE (Biomarker-integrated Approaches of Targeted Therapy for Lung Cancer Elimination) trial applied an adaptive approach to match lung cancer patients with targeted therapies based on their specific biomarkers. By continuously analyzing patient responses and adjusting treatment regimens accordingly, the BATTLE trial demonstrated how adaptive designs could personalize therapy and improve outcomes for cancer patients.
These examples illustrate the transformative potential of adaptive clinical trials. By enhancing flexibility, efficiency, and cost-effectiveness, adaptive trials are paving the way for more responsive and patient-centered clinical research, ultimately leading to faster and more accurate development of new treatments.
Decentralized Clinical Trials (DCTs)
Definition and Components of Decentralized Trials
Decentralized Clinical Trials (DCTs) represent a revolutionary shift in the way clinical research is conducted. Unlike traditional trials, which typically require participants to visit centralized sites such as hospitals or clinics, DCTs utilize digital technologies to conduct studies remotely. This approach allows for participant recruitment, data collection, and monitoring to occur outside of traditional clinical settings, often from the participants' homes. Components of DCTs include telemedicine consultations, remote patient monitoring, electronic data capture, and home-based healthcare services.
Advantages: Increased Participant Diversity, Improved Patient Retention, Reduced Logistical Barriers
- Increased Participant Diversity: DCTs can reach a broader and more diverse participant pool by eliminating geographical constraints. This inclusivity is particularly beneficial for enrolling underrepresented populations, including those from rural or underserved areas. By ensuring a more diverse participant base, DCTs can enhance the generalizability of trial results.
- Improved Patient Retention: The convenience of participating from home or a local facility often leads to higher patient retention rates in DCTs. Participants are less likely to drop out due to the burdens of travel, time, or scheduling conflicts. This retention is crucial for maintaining the integrity and validity of clinical trial data.
- Reduced Logistical Barriers: DCTs reduce many logistical challenges associated with traditional trials. By leveraging digital tools and remote monitoring, these trials can minimize the need for physical site visits, streamline data collection, and expedite the overall trial process. This efficiency not only saves time but also reduces costs associated with site management and patient travel.
Examples of Technologies Enabling DCTs
1. Telemedicine: Telemedicine platforms facilitate virtual consultations between participants and healthcare providers. These platforms enable remote assessment, monitoring, and follow-up, making it easier to conduct clinical trials without the need for in-person visits. Telemedicine has been particularly crucial during the COVID-19 pandemic, allowing trials to continue despite social distancing requirements.
2. Wearable Devices: Wearable health devices, such as smartwatches and fitness trackers, play a significant role in DCTs by continuously monitoring vital signs, activity levels, and other health metrics. These devices provide real-time data that can be transmitted directly to researchers, offering a comprehensive view of a participant's health and treatment response.
3. Electronic Data Capture (EDC) Systems: EDC systems enable the secure collection and storage of trial data electronically. These systems streamline data entry, reduce errors, and facilitate real-time data analysis. EDC systems are essential for managing the large volumes of data generated in DCTs efficiently.
4. Home-Based Healthcare Services: Some DCTs incorporate home-based healthcare services, such as mobile phlebotomy or nursing visits, to collect samples or administer treatments. These services ensure that participants receive the necessary medical support without needing to visit a clinical site, further enhancing the convenience and feasibility of DCTs.
Case Study: The REMOTE Trial
A notable example of a successful DCT is the REMOTE trial conducted by Pfizer. This trial aimed to evaluate the feasibility of a fully remote trial design by studying the efficacy of a new overactive bladder treatment. The REMOTE trial utilized online recruitment, telemedicine consultations, and electronic data capture to conduct the study. Despite facing some challenges, the trial demonstrated the potential of DCTs to reduce costs and improve patient convenience without compromising data integrity.
Real-World Evidence (RWE) and Real-World Data (RWD)
Explanation of RWE and RWD
Real-World Evidence (RWE) and Real-World Data (RWD) are transforming the clinical research landscape by providing insights derived from everyday clinical practice. RWD encompasses data collected outside of traditional clinical trials, such as electronic health records (EHRs), claims and billing activities, product and disease registries, patient-generated data including in-home use settings, and data gathered from other sources that can inform on health status, such as mobile devices. RWE is the clinical evidence derived from the analysis and interpretation of RWD. It reflects the performance and effectiveness of medical interventions in a broader, more varied patient population than those typically involved in clinical trials.
How RWE and RWD are Integrated into Clinical Trials
1. Study Design Enhancement: By incorporating RWD, researchers can design more inclusive and pragmatic trials that better reflect real-world clinical settings. RWE can help identify patient populations that are more likely to benefit from the intervention, thereby refining inclusion and exclusion criteria.
2. Data Collection and Analysis: RWD can be used alongside traditional clinical trial data to provide a more comprehensive view of treatment outcomes. For example, wearable devices and mobile health apps can continuously collect patient data, supplementing the data obtained during scheduled trial visits.
3. Monitoring and Safety Assessments: RWE can provide ongoing safety data that complement clinical trial findings. Continuous monitoring of real-world patient outcomes can help detect adverse events earlier and in a broader population.
4. Post-Market Surveillance: RWE is crucial for post-market surveillance, allowing researchers to track the long-term safety and effectiveness of treatments once they are in widespread use. This ongoing data collection can identify rare side effects or long-term benefits that may not have been apparent in pre-approval trials.
Impact on Drug Development and Regulatory Approvals
1. Faster and More Informed Decision-Making: The integration of RWE into the drug development process allows for more rapid and informed decision-making. By providing a broader context of how treatments perform in everyday settings, RWE can accelerate the identification of promising therapies and expedite their progression through the development pipeline.
2. Enhanced Regulatory Submissions: Regulatory agencies, such as the FDA and EMA, are increasingly recognizing the value of RWE in supporting drug approvals. RWE can supplement traditional clinical trial data, offering additional evidence of a treatment's effectiveness and safety. This supplementary data can be particularly valuable in cases where traditional trials are impractical or ethically challenging.
3. Adaptive Pathways and Conditional Approvals: RWE can support adaptive pathways and conditional approvals by providing real-time data that can be used to refine and adjust treatment use in clinical practice. These approaches allow for earlier access to promising therapies while continuing to gather evidence on their effectiveness and safety.
4. Improved Patient Outcomes: Ultimately, the use of RWE and RWD in clinical research can lead to better patient outcomes. By providing a more comprehensive understanding of how treatments work in diverse, real-world populations, RWE can guide personalized medicine approaches, ensuring that the right patients receive the right treatments at the right time.
Case Study: The Use of RWE in Oncology
In oncology, the use of RWE has been particularly impactful. The FDA's approval of the drug palbociclib for breast cancer was supported by RWE derived from electronic health records and insurance claims data. This evidence demonstrated the drug's effectiveness and safety in a real-world setting, complementing the data from clinical trials and supporting the regulatory decision. This case highlights how RWE can bridge the gap between clinical trial data and real-world clinical practice, ultimately leading to faster access to effective treatments for patients.
The integration of Real-World Evidence and Real-World Data into clinical research is a powerful trend that is reshaping drug development and regulatory processes. By providing insights that are more reflective of everyday clinical practice, RWE and RWD enhance the relevance and applicability of clinical research, ultimately driving improvements in patient care and treatment outcomes.
An exemplary role model is Notable Labs which is at the forefront of clinical research, leveraging its Predictive Precision Medicine Platform (PPMP) to enhance drug development. Recent activities include the design plan for a Phase 2 trial with Volasertib for relapsed/refractory AML, innovations in using cryopreserved samples in the PPMP assay, and a partnership with the MDS Foundation to advance drug therapies. Their efforts focus on integrating AI and real-world outcomes to predict trial results accurately and improve patient-specific treatments.
Artificial Intelligence (AI) and Machine Learning (ML) in Clinical Research
Overview of AI and ML Applications in Clinical Trials
Artificial Intelligence (AI) and Machine Learning (ML) are revolutionizing clinical research by providing powerful tools to handle complex data, identify patterns, and predict outcomes. AI refers to the simulation of human intelligence in machines, while ML is a subset of AI that involves algorithms learning from and making predictions based on data. In clinical trials, AI and ML are applied across various stages, including study design, patient recruitment, data collection and analysis, and monitoring.
Examples of AI/ML Improving Data Analysis, Patient Recruitment, and Trial Monitoring
1. Data Analysis:
AI and ML can process vast amounts of clinical data far more quickly and accurately than traditional methods. These technologies can identify patterns and correlations within data that might be missed by human analysis. For instance, ML algorithms can analyze genomic data to identify biomarkers predictive of treatment response, leading to more targeted and effective therapies.
Example: IBM Watson for Clinical Trial Matching utilizes AI to analyze patient data and match patients with appropriate clinical trials. This system significantly speeds up the process of finding suitable trials, ensuring that patients receive timely access to potentially life-saving treatments.
2. Patient Recruitment:
AI and ML can enhance patient recruitment by analyzing electronic health records, social media activity, and other data sources to identify potential trial participants. These technologies can match patients with trials based on their medical history, genetic profile, and other relevant criteria.
Example: Deep6 AI uses machine learning to sift through unstructured data in medical records, identifying patients who meet specific clinical trial criteria. This tool has reduced the time required for patient recruitment from months to minutes, significantly accelerating the trial initiation process.
3. Trial Monitoring:
AI and ML enable continuous and real-time monitoring of clinical trials. These technologies can detect anomalies, predict adverse events, and provide early warnings of potential safety issues. This proactive approach helps ensure patient safety and data integrity throughout the trial.
Example: Medidata's Sensor Cloud platform leverages AI to integrate and analyze data from wearable devices and other digital health technologies. This platform provides real-time insights into patient health, allowing for timely interventions and more accurate assessments of treatment efficacy.
Challenges and Considerations in Implementing AI/ML
Data Quality and Integration:
The effectiveness of AI and ML depends on the quality and completeness of the data they analyze. Inconsistent, incomplete, or biased data can lead to inaccurate predictions and analyses. Ensuring high-quality, standardized data from diverse sources is a significant challenge.
Regulatory and Ethical Concerns:
The use of AI and ML in clinical trials raises important regulatory and ethical questions. Regulatory bodies need to establish clear guidelines for the use of these technologies to ensure patient safety and data privacy. Additionally, ethical considerations related to algorithmic bias and the transparency of AI decision-making processes must be addressed.
Interoperability:
Integrating AI and ML tools with existing clinical trial systems and databases can be complex. Ensuring interoperability between different technologies and platforms is crucial for seamless data exchange and analysis.
Skill Gaps:
Implementing AI and ML in clinical research requires specialized skills in data science and machine learning. There is a growing need for professionals who can bridge the gap between clinical research and advanced data analytics.
Cost and Resource Allocation:
Developing and deploying AI and ML technologies can be costly. Organizations must balance the investment in these technologies with the potential benefits, ensuring that resources are allocated effectively to achieve the desired outcomes.
Case Study: AI in Drug Discovery
One notable example of AI's impact on clinical research is its application in drug discovery. Insilico Medicine, an AI-driven biotechnology company, uses deep learning algorithms to identify new drug candidates. Their AI system analyzed vast amounts of biological data to propose novel drug molecules, significantly reducing the time and cost associated with traditional drug discovery methods. One of their AI-discovered drug candidates progressed from initial discovery to preclinical testing in less than two years, showcasing the transformative potential of AI in accelerating drug development.
The integration of Artificial Intelligence and Machine Learning into clinical research is poised to enhance the efficiency, accuracy, and speed of clinical trials. By addressing the challenges and leveraging the full potential of these technologies, the clinical research industry can move towards a future where data-driven insights lead to more effective treatments and improved patient outcomes.
Precision Medicine and Personalized Trials
Definition of Precision Medicine and Its Relevance to Clinical Research
Precision medicine is an innovative approach to medical treatment that takes into account individual variability in genes, environment, and lifestyle for each person. This approach contrasts with the traditional "one-size-fits-all" model, where treatments are designed for the average patient. Precision medicine aims to tailor treatments to individual patients based on their unique genetic and molecular profiles, leading to more effective and targeted therapies.
In clinical research, precision medicine is particularly relevant as it allows for the development of treatments that are specifically designed to work best for particular subgroups of patients. This focus on individual differences can lead to better outcomes, fewer side effects, and a more efficient drug development process. Precision medicine is driving the evolution of clinical trials towards more personalized approaches, ensuring that the right patients receive the right treatments at the right time.
How Personalized Trials Are Designed and Conducted
Patient Stratification:
Personalized trials begin with the stratification of patients based on specific biomarkers or genetic profiles. Advanced diagnostic tools and genomic sequencing are used to identify these biomarkers, which can predict how patients will respond to a particular treatment. This stratification allows researchers to design trials that target specific patient subgroups more likely to benefit from the intervention.
Adaptive Trial Designs:
Personalized trials often use adaptive trial designs, where interim data analysis allows for modifications to the study protocol. For example, if a particular subgroup shows a significant response to the treatment, the trial can be adapted to focus more on that subgroup, optimizing resources and increasing the likelihood of success.
Biomarker-Driven Selection:
In personalized trials, patients are selected based on their biomarker profiles. This selection process ensures that only those patients most likely to respond to the treatment are enrolled, enhancing the trial’s efficiency and effectiveness. Biomarker-driven selection is particularly important in oncology, where targeted therapies can significantly improve patient outcomes.
Real-Time Monitoring and Adjustments:
Personalized trials utilize real-time monitoring to track patient responses and adjust treatment plans as needed. This approach allows for immediate interventions if a patient is not responding well to the treatment, ensuring patient safety and optimizing therapeutic outcomes.
Examples of Precision Medicine Improving Trial Outcomes
Herceptin (Trastuzumab) for Breast Cancer:
Herceptin is a targeted therapy for HER2-positive breast cancer, a subtype that overexpresses the HER2 protein. Clinical trials for Herceptin involved selecting patients based on their HER2 status, leading to significantly improved outcomes for those with HER2-positive tumors. This targeted approach has made Herceptin a cornerstone of precision medicine in oncology.
Keytruda (Pembrolizumab) for Various Cancers:
Keytruda is an immunotherapy drug that targets the PD-1 pathway, used to treat various cancers. Clinical trials for Keytruda have employed precision medicine strategies by selecting patients based on their PD-L1 expression levels. This biomarker-driven approach has led to remarkable success in treating cancers such as melanoma, lung cancer, and more, demonstrating the power of personalized trials in improving patient outcomes.
PARP Inhibitors for BRCA-Mutated Cancers:
PARP inhibitors, such as Olaparib, are used to treat cancers with BRCA1 or BRCA2 mutations. Clinical trials for these drugs focused on patients with these specific genetic mutations, leading to substantial improvements in progression-free survival and overall survival rates. This precision medicine approach has transformed the treatment landscape for patients with BRCA-mutated cancers.
Case Study: NCI-MATCH Trial
The NCI-MATCH (Molecular Analysis for Therapy Choice) trial is a groundbreaking example of a precision medicine clinical trial. This trial involves sequencing the tumors of patients with various cancers to identify genetic mutations and match them with targeted therapies. Patients are assigned to treatment arms based on their specific genetic alterations, regardless of their cancer type. The NCI-MATCH trial has demonstrated the feasibility and effectiveness of precision medicine, providing valuable insights into how targeted therapies can be matched to individual patients to improve outcomes.
Precision medicine and personalized trials are revolutionizing clinical research by focusing on individual patient characteristics to develop targeted therapies. By designing trials that consider genetic, environmental, and lifestyle differences, researchers can achieve more precise and effective treatments, ultimately leading to better patient outcomes and a more efficient drug development process.
Patient-Centric Approaches
The Shift Towards Patient-Centric Trial Designs
The landscape of clinical trials is increasingly shifting towards patient-centric approaches, placing patients at the heart of the research process. Traditional trials often focus more on the needs of researchers and sponsors, sometimes at the expense of participant convenience and comfort. In contrast, patient-centric trial designs prioritize the experiences, preferences, and outcomes of patients, aiming to make participation more accessible, engaging, and relevant to their daily lives. This shift is driven by the recognition that patient engagement and satisfaction are crucial for the success of clinical trials, influencing recruitment, retention, and overall data quality.
Methods for Improving Patient Engagement and Compliance
Simplified Consent Processes:
The informed consent process can be complex and overwhelming for many patients. Simplifying consent forms, using plain language, and incorporating multimedia tools such as videos and interactive modules can make the process more understandable and less intimidating, leading to higher engagement and consent rates.
Flexible Study Designs:
Offering flexible participation options, such as virtual visits, home-based interventions, and flexible scheduling, can accommodate patients’ lifestyles and reduce the burden of participation. This flexibility can improve compliance and retention by making it easier for patients to integrate trial activities into their daily routines.
Enhanced Communication:
Regular, clear, and empathetic communication between researchers and participants is essential for maintaining engagement. Providing updates on trial progress, addressing concerns promptly, and offering support through various communication channels (e.g., phone, email, mobile apps) can strengthen the patient-researcher relationship and encourage ongoing participation.
Patient Education and Empowerment:
Educating patients about the importance of the trial and their role in it can foster a sense of ownership and responsibility. Providing educational materials and resources that explain the trial’s purpose, procedures, and potential benefits can empower patients to actively participate and adhere to the study protocols.
Incorporating Patient Feedback:
Actively seeking and incorporating patient feedback throughout the trial process can improve the trial design and patient experience. Surveys, focus groups, and patient advisory boards can provide valuable insights into patient needs and preferences, helping to tailor the trial to better meet those needs.
Case Studies of Patient-Centric Trials
1. The Apple Heart Study:
The Apple Heart Study, conducted by Stanford Medicine in collaboration with Apple, is a prime example of a patient-centric trial. This study aimed to identify irregular heart rhythms using the Apple Watch’s heart rate sensor. The trial design allowed participants to enroll through a mobile app, monitor their heart health remotely, and receive notifications if an irregular rhythm was detected. The study’s user-friendly, technology-driven approach resulted in high participant engagement and compliance, demonstrating the potential of digital health tools in patient-centric research.
2. The Parkinson’s Disease Digital Health Study:
The Parkinson’s Disease Digital Health Study, led by Roche and using digital health technologies, aimed to monitor and analyze the symptoms of Parkinson’s disease in real-time. Patients used wearable devices and a mobile app to track their symptoms and medication adherence. The study’s design focused on minimizing the burden on participants by allowing data collection in their natural environment. This patient-centric approach provided continuous, real-world data and improved patient engagement by integrating the trial into their daily lives.
3. The REMOTE Trial by Pfizer:
The REMOTE trial by Pfizer was one of the first fully virtual clinical trials, focusing on an overactive bladder treatment. Participants enrolled online, completed electronic consent, and reported outcomes through digital tools. The trial’s decentralized, patient-centric design eliminated the need for site visits, making participation more convenient and accessible. Although the trial faced challenges, it highlighted the potential of virtual trials to enhance patient engagement and reduce logistical barriers.
Use of Biomarkers and Genomics
Role of Biomarkers and Genomics in Modern Clinical Trials
Biomarkers and genomics play a crucial role in modern clinical trials, driving the advancement of personalized medicine and targeted therapies. Biomarkers are biological indicators that can be measured to assess health conditions, disease progression, or responses to treatments. They can include proteins, genes, metabolites, or other molecules found in blood, tissues, or other bodily fluids. Genomics, the study of an organism's complete set of DNA, including all of its genes, provides a comprehensive understanding of genetic variations and their impact on health and disease.
In clinical trials, biomarkers and genomic data are used to:
- Identify and select patient populations likely to benefit from a specific treatment.
- Monitor treatment responses and disease progression in real time.
- Predict adverse reactions or resistance to therapies.
- Enhance the understanding of disease mechanisms and drug action.
How They Contribute to More Targeted and Effective Treatments
Patient Stratification and Selection:
Biomarkers and genomic data enable the stratification of patients based on their molecular profiles. By identifying specific genetic mutations, protein expressions, or other biomarkers, researchers can select patients who are more likely to respond to a particular treatment. This targeted approach increases the likelihood of success and reduces the exposure of non-responders to potentially ineffective treatments.
Personalized Treatment Plans:
The integration of biomarkers and genomics allows for the development of personalized treatment plans tailored to the individual characteristics of each patient. Personalized therapies can lead to better outcomes by addressing the underlying causes of the disease at a molecular level.
Improved Efficacy and Safety:
Biomarker-driven trials can lead to improved efficacy by focusing on patient populations with a higher probability of response. Additionally, identifying biomarkers associated with adverse reactions can enhance safety by excluding patients at risk of severe side effects.
Real-Time Monitoring and Adaptation:
Biomarkers and genomic data facilitate real-time monitoring of treatment responses, enabling early detection of changes in disease status. This capability allows for timely adjustments to treatment plans, improving overall outcomes and patient management.
Examples of Trials Leveraging Biomarkers and Genomic Data
The BATTLE Trial:
The BATTLE (Biomarker-integrated Approaches of Targeted Therapy for Lung Cancer Elimination) trial used biomarker profiles to guide the treatment of non-small cell lung cancer (NSCLC) patients. By analyzing biomarkers such as EGFR mutations and KRAS mutations, researchers assigned patients to targeted therapies tailored to their specific molecular characteristics. This approach led to improved treatment responses and demonstrated the feasibility of biomarker-driven personalized medicine in oncology.
The IMPACT Study:
The IMPACT (Identification of Men with a Genetic Predisposition to Prostate Cancer) study focused on men with BRCA1 and BRCA2 mutations to evaluate the effectiveness of targeted screening and early detection of prostate cancer. By leveraging genetic biomarkers, the study identified high-risk individuals and provided personalized screening recommendations, leading to earlier diagnosis and better outcomes.
NCI-MATCH Trial:
The NCI-MATCH (Molecular Analysis for Therapy Choice) trial is a landmark study that matches patients with targeted therapies based on their specific genetic alterations. Tumor samples from patients with various cancer types are sequenced to identify actionable mutations. Participants are then assigned to treatment arms with drugs designed to target their unique genetic profiles. This innovative approach has provided valuable insights into the effectiveness of targeted therapies across different cancers and highlighted the potential of precision medicine.
The TAILORx Trial:
The TAILORx (Trial Assigning IndividuaLized Options for Treatment) trial in breast cancer utilized a genomic test called Oncotype DX to assess the expression of 21 genes associated with cancer recurrence. Based on the test results, patients with early-stage breast cancer were stratified into different treatment groups, receiving either hormone therapy alone or in combination with chemotherapy. The trial demonstrated that many women with low to intermediate risk scores could avoid chemotherapy without compromising their outcomes, highlighting the role of genomic testing in personalizing treatment plans.
Remote Monitoring and Digital Health Technologies
Technologies Enabling Remote Monitoring in Clinical Trials
Remote monitoring technologies are transforming the way clinical trials are conducted by enabling continuous, real-time data collection from participants outside traditional clinical settings. These technologies include:
Wearable Devices:
Devices such as smartwatches, fitness trackers, and biosensors can monitor various health metrics, including heart rate, physical activity, sleep patterns, and more. Examples include the Apple Watch, Fitbit, and devices from companies like Garmin and BioIntelliSense.
Mobile Health Applications:
Mobile apps facilitate the collection of patient-reported outcomes, medication adherence, and symptom tracking. These apps can also provide reminders and educational content to support patient engagement and compliance.
Telemedicine Platforms:
Telemedicine platforms enable virtual consultations and remote assessments, allowing researchers to interact with participants without the need for in-person visits. Platforms like Teladoc, Amwell, and Zoom for Healthcare have become integral to remote clinical trials.
Remote Diagnostic Tools:
Tools such as digital stethoscopes, portable ECG monitors, and home lab test kits enable participants to conduct medical tests and share results with researchers from their homes. Companies like AliveCor and Eko provide these types of diagnostic devices.
Electronic Data Capture (EDC) Systems:
EDC systems facilitate the secure and efficient collection and management of trial data. These systems often integrate with wearable devices and mobile apps to streamline data entry and analysis.
Benefits of Remote Monitoring for Patient Safety and Data Accuracy
Enhanced Patient Safety:
Continuous monitoring allows for the early detection of adverse events and health changes, enabling timely interventions and reducing the risk of serious complications. Real-time data can alert researchers to potential issues before they become critical.
Improved Data Accuracy:
Remote monitoring technologies provide objective, real-time data that reduce reliance on patient recall and self-reporting, which can be prone to inaccuracies. The continuous nature of data collection also provides a more comprehensive picture of a participant's health status.
Increased Patient Engagement and Compliance:
The convenience of remote monitoring can enhance patient engagement and adherence to study protocols. Participants can easily integrate monitoring into their daily routines, reducing the burden of frequent site visits and increasing overall trial retention rates.
Broader Participant Recruitment:
Remote monitoring enables the inclusion of participants from diverse geographic locations, including those in rural or underserved areas. This inclusivity enhances the generalizability of trial results by representing a wider population.
Examples of Digital Health Tools Used in Trials
Verily's Project Baseline:
Verily's Project Baseline uses a combination of wearable devices, mobile apps, and home-based diagnostic tools to collect comprehensive health data from participants. The project aims to map human health and identify early signals of disease. Participants use devices such as the Study Watch to monitor vital signs and participate in virtual visits through the Baseline app.
The Apple Heart Study:
Conducted by Stanford Medicine and Apple, the Apple Heart Study used the Apple Watch to detect irregular heart rhythms. Participants enrolled via a mobile app and received notifications if the watch detected an irregular rhythm. The study demonstrated the feasibility and effectiveness of using wearable devices for large-scale, remote clinical research.
The mHealth Screening to Prevent Strokes (mSToPS) Trial:
The mSToPS trial, led by Scripps Translational Science Institute, evaluated the use of the iRhythm Zio XT patch, a wearable ECG monitor, to screen for atrial fibrillation. Participants wore the patch at home, allowing researchers to remotely monitor their heart rhythms and identify asymptomatic atrial fibrillation cases. The trial showed that remote monitoring could effectively screen for and detect atrial fibrillation in a real-world setting.
The REMOTE Trial by Pfizer:
The REMOTE trial, one of the first fully virtual clinical trials, used digital health technologies to study an overactive bladder treatment. Participants enrolled online, provided consent electronically, and reported outcomes via digital tools. The trial utilized wearable devices and mobile apps to collect data, eliminating the need for site visits and demonstrating the potential of decentralized trials.
Regulatory Innovations and Fast-Track Approvals
Overview of Recent Regulatory Changes Affecting Clinical Research
Regulatory bodies worldwide have recognized the need to adapt and innovate to keep pace with the rapid advancements in medical research and technology. Recent changes in regulatory frameworks aim to streamline the clinical trial process, reduce the time to market for new therapies, and ensure patient safety. These changes include:
Accelerated Approval Programs:
Agencies like the FDA and EMA have implemented programs such as Fast Track, Breakthrough Therapy, Accelerated Approval, and Priority Review to expedite the development and review of drugs that address unmet medical needs or show substantial improvement over existing therapies.
Adaptive Trial Designs:
Regulators have shown increased acceptance of adaptive trial designs, which allow modifications to the trial protocol based on interim data. This flexibility can lead to more efficient trials and faster conclusions.
Real-World Evidence (RWE) Integration:
Regulatory bodies are increasingly considering RWE to support drug approvals and post-market surveillance. This approach can provide additional data on a drug’s safety and effectiveness in a broader, real-world population.
Use of Digital Health Technologies:
Regulatory agencies are providing guidance on the use of digital health technologies, such as wearables and telemedicine, in clinical trials. This includes ensuring data integrity, patient privacy, and the validation of digital endpoints.
Impact of Fast-Track Approvals and Breakthrough Designations
Reduced Time to Market:
Fast-track approvals and breakthrough designations significantly reduce the time required for a drug to move from development to market. This is particularly important for therapies targeting serious or life-threatening conditions, where patients may have limited treatment options.
Increased Investment in Innovation:
The promise of expedited review and approval processes encourages pharmaceutical companies to invest in innovative treatments. Knowing that a potential therapy could reach patients faster provides a strong incentive to pursue cutting-edge research.
Early Access for Patients:
Patients gain earlier access to potentially life-saving treatments. For conditions with limited or no treatment options, fast-track approvals can provide a crucial lifeline.
Enhanced Collaboration:
Fast-track and breakthrough pathways often involve increased collaboration between regulatory agencies and sponsors. This ongoing dialogue can help identify and resolve issues early in the development process, leading to more efficient trials.
Examples of Drugs/Therapies that Benefited from Regulatory Innovations
Keytruda (Pembrolizumab):
Keytruda, an immunotherapy drug used to treat various cancers, received multiple breakthrough therapy designations and accelerated approvals from the FDA. This expedited process allowed Keytruda to reach the market more quickly, providing a new treatment option for patients with melanoma, lung cancer, and other cancers.
Spinraza (Nusinersen):
Spinraza, a treatment for spinal muscular atrophy (SMA), benefited from priority review and breakthrough therapy designation. These regulatory pathways facilitated a faster approval process, enabling Spinraza to become the first approved treatment for SMA and significantly improving outcomes for patients with this rare genetic disorder.
Veklury (Remdesivir):
Veklury, an antiviral drug used to treat COVID-19, was granted emergency use authorization (EUA) by the FDA, allowing its use during the pandemic based on preliminary data. The EUA was later followed by full approval, demonstrating how regulatory flexibility can address urgent public health needs.
Zolgensma (Onasemnogene Abeparvovec):
Zolgensma, a gene therapy for spinal muscular atrophy, received breakthrough therapy designation and priority review. These regulatory innovations enabled a swift approval process, making Zolgensma available to patients much sooner and offering a one-time treatment option with transformative potential.
Summary of the key trends and their significance
In recent years, several key trends have emerged in clinical research methodologies, each contributing to the transformation of the field. These trends include:
1. Adaptive Clinical Trials:
Adaptive trials introduce flexibility by allowing modifications to the study design based on interim results. This approach enhances efficiency, reduces costs, and increases the likelihood of identifying effective treatments sooner.
2. Decentralized Clinical Trials (DCTs):
DCTs leverage digital technologies to conduct studies remotely, improving participant diversity, engagement, and retention. This model reduces logistical barriers and makes trials more accessible to a broader population.
3. Real-World Evidence (RWE) and Real-World Data (RWD):
The integration of RWE and RWD into clinical trials offers a more comprehensive understanding of treatment effectiveness and safety in real-world settings. This data can support regulatory decisions and improve post-market surveillance.
4. Artificial Intelligence (AI) and Machine Learning (ML):
AI and ML enhance data analysis, patient recruitment, and trial monitoring by identifying patterns, predicting outcomes, and optimizing processes. These technologies drive efficiency and precision in clinical research.
5. Precision Medicine and Personalized Trials:
Precision medicine tailors treatments based on individual genetic and molecular profiles, leading to more targeted and effective therapies. Personalized trials enhance patient outcomes by focusing on specific subgroups likely to benefit from the intervention.
6. Patient-Centric Approaches:
Patient-centric trial designs prioritize the experiences and needs of participants, improving engagement, compliance, and retention. These approaches ensure that trials are more relevant and accessible to patients.
7. Use of Biomarkers and Genomics:
Biomarkers and genomics enable the identification of patient subgroups, real-time monitoring, and the development of targeted therapies. This focus on molecular profiles enhances the precision and effectiveness of clinical research.
8. Remote Monitoring and Digital Health Technologies:
Remote monitoring tools facilitate continuous data collection, improving patient safety and data accuracy. These technologies support decentralized trials and enhance the overall efficiency of clinical research.
9. Regulatory Innovations and Fast-Track Approvals:
Regulatory bodies have introduced programs to expedite the development and review of new therapies, ensuring that patients gain access to effective treatments more quickly. These innovations encourage investment in novel research and support the rapid advancement of medical science.
The Future Outlook for Clinical Research Methodologies
The future of clinical research methodologies looks promising, with continued advancements and innovations poised to reshape the field. Key areas of focus will likely include:
Integration of Advanced Technologies:
The use of AI, ML, digital health tools, and remote monitoring will continue to grow, enhancing the efficiency, precision, and patient-centricity of clinical trials.
Expansion of Personalized Medicine:
Personalized and precision medicine will become more prevalent, with trials increasingly designed to target specific patient subgroups based on genetic and molecular profiles.
Enhanced Regulatory Frameworks:
Regulatory agencies will further refine and adapt their frameworks to accommodate new methodologies and technologies, ensuring that the approval process remains rigorous yet flexible.
Increased Patient Involvement:
Patient engagement will remain a priority, with more trials incorporating patient feedback and preferences to improve the overall trial experience and outcomes.
Global Collaboration:
International collaboration will expand, enabling the sharing of data, resources, and expertise across borders. This collaboration will drive more comprehensive and diverse clinical research.
The continuous evolution of clinical research methodologies reflects the dynamic nature of the field and its commitment to improving patient outcomes. As new technologies and approaches emerge, clinical research will become more efficient, precise, and patient-centric. These advancements will not only accelerate the development of new therapies but also ensure that treatments are safer, more effective, and tailored to the needs of individual patients.
The integration of adaptive trial designs, decentralized models, real-world data, AI, and precision medicine represents a paradigm shift in how clinical research is conducted. By embracing these innovations, the clinical research community can address the challenges of traditional methodologies and pave the way for a future where medical advancements are achieved faster and more effectively.
In conclusion, the ongoing evolution of clinical research methodologies promises to enhance the quality and impact of clinical trials, ultimately benefiting patients worldwide. As researchers, regulators, and healthcare providers continue to innovate and collaborate, the future of clinical research holds immense potential for advancing medical science and improving global health outcomes.
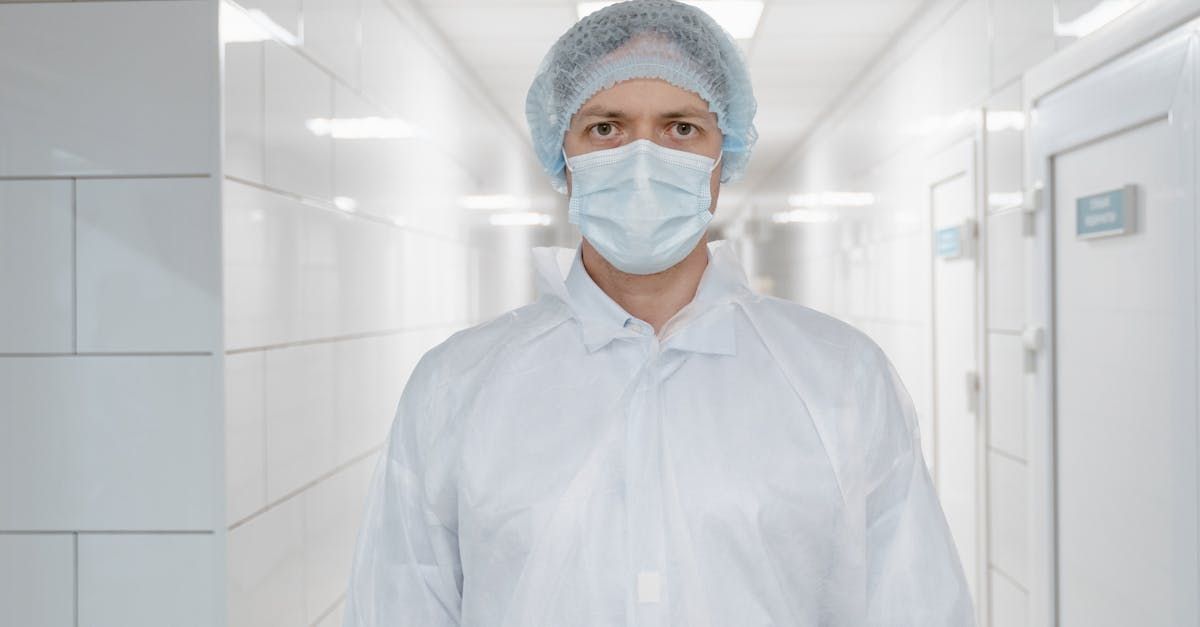
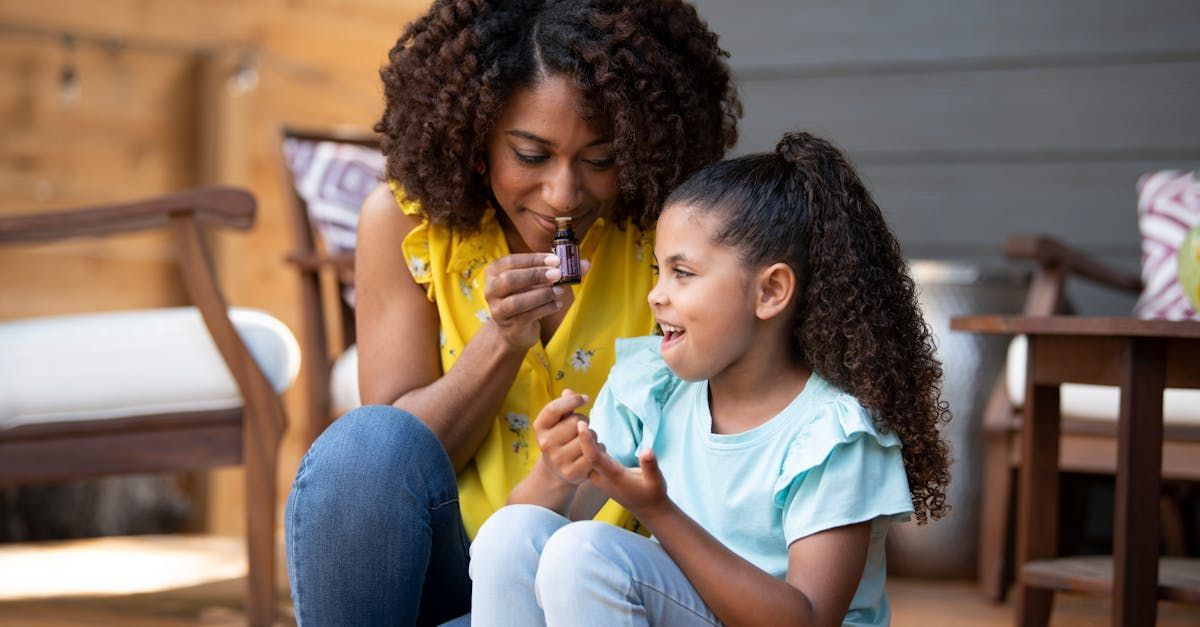
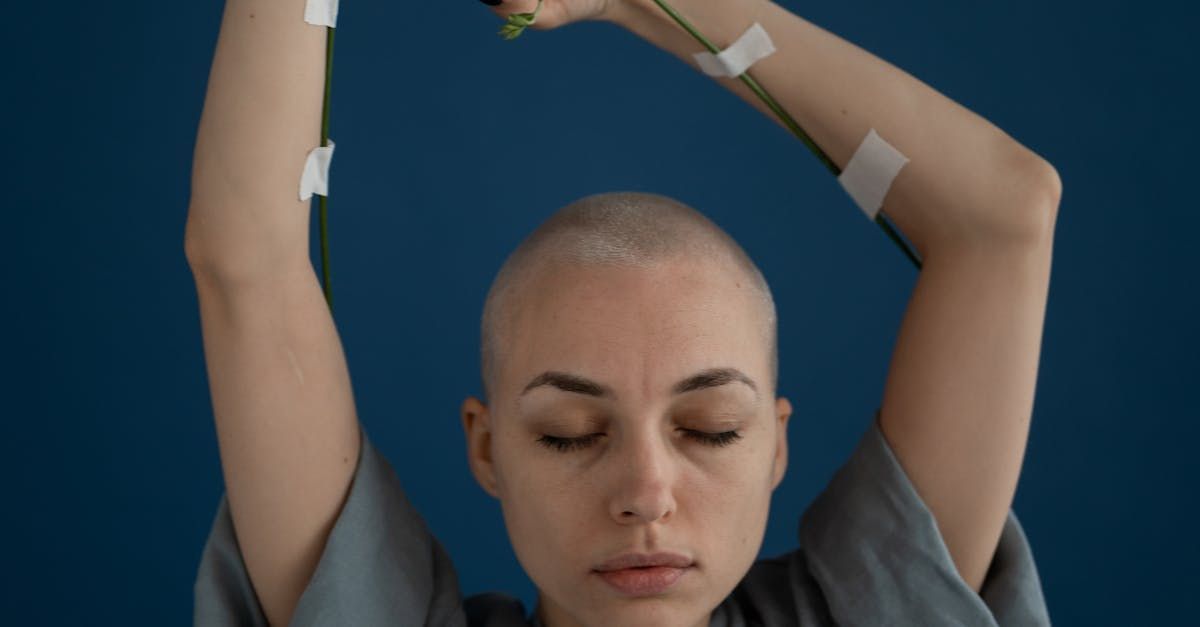
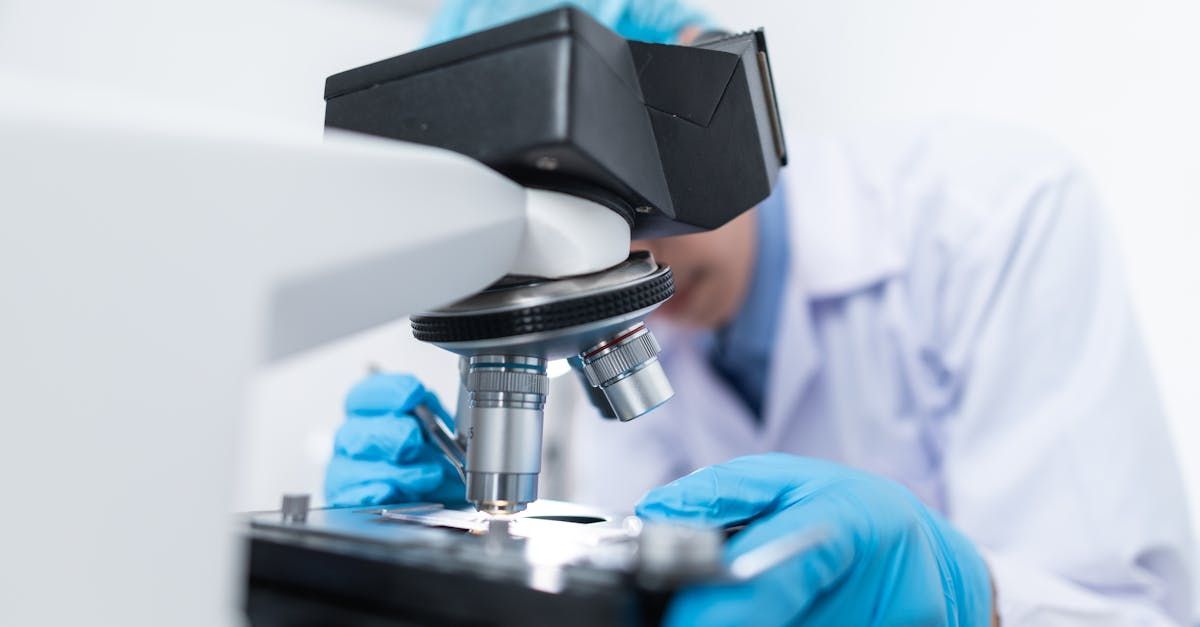
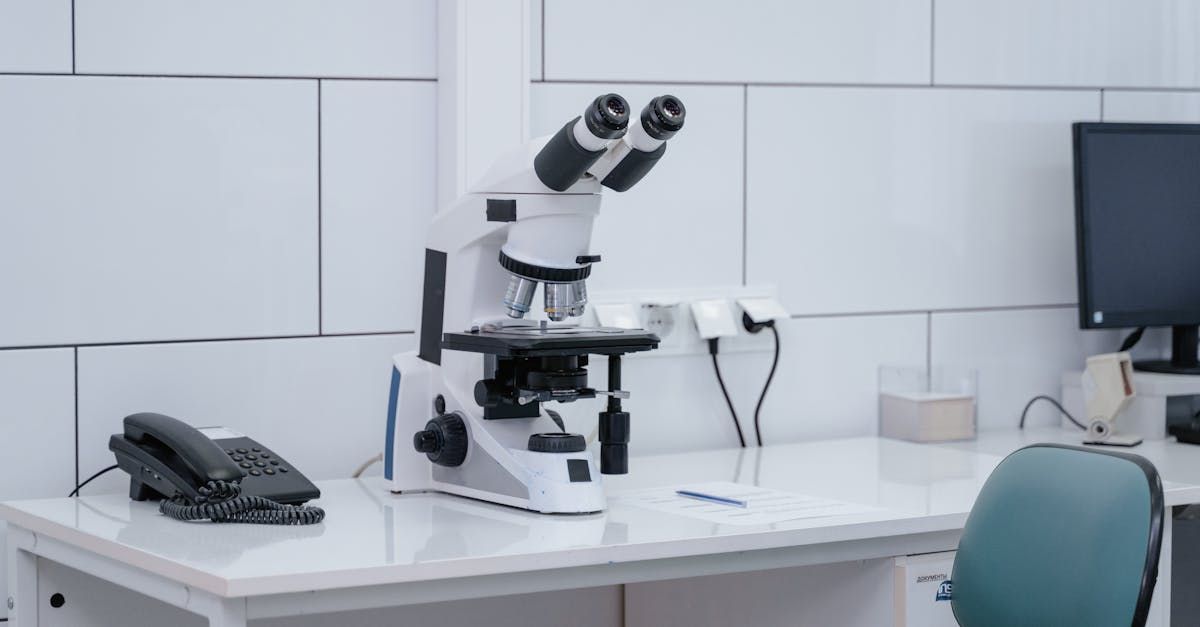
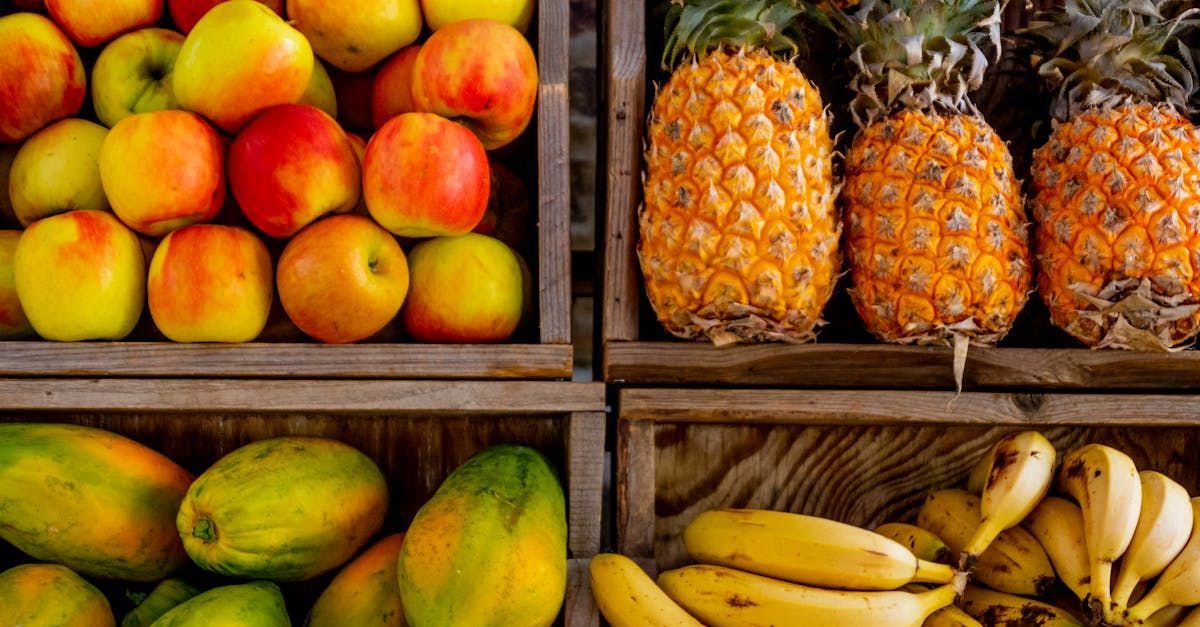
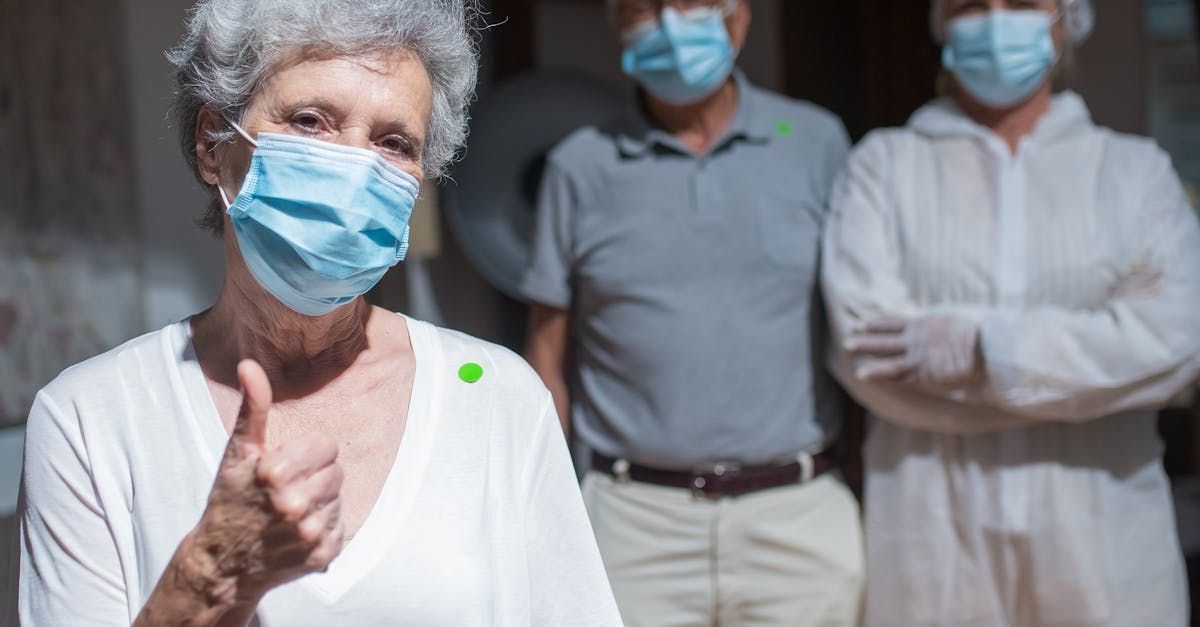
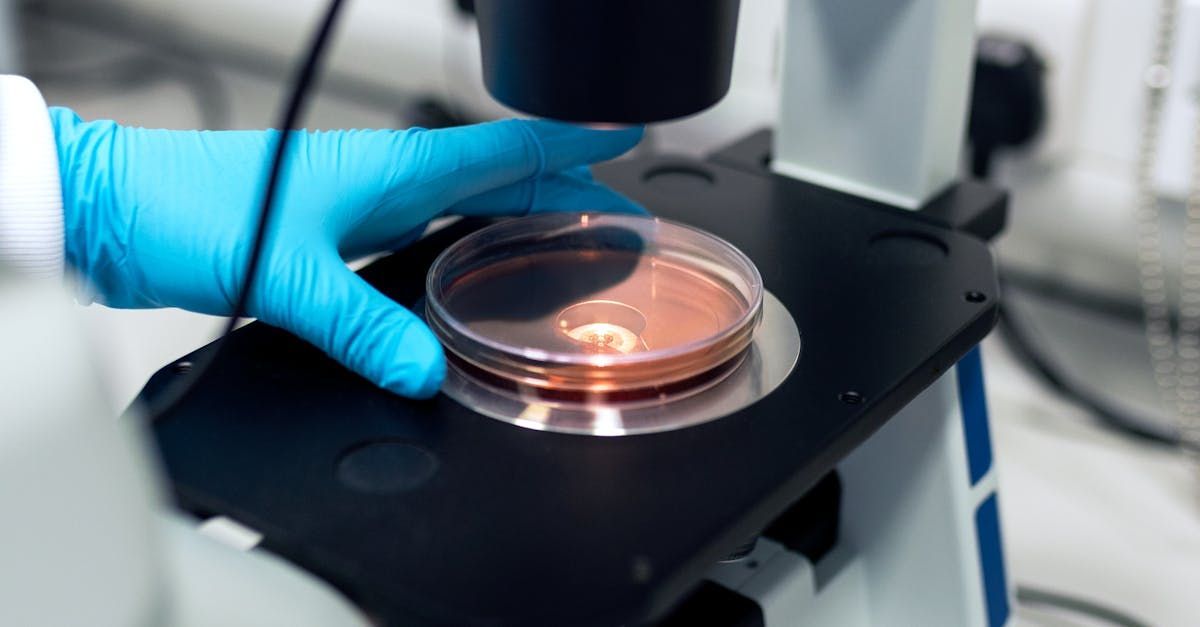
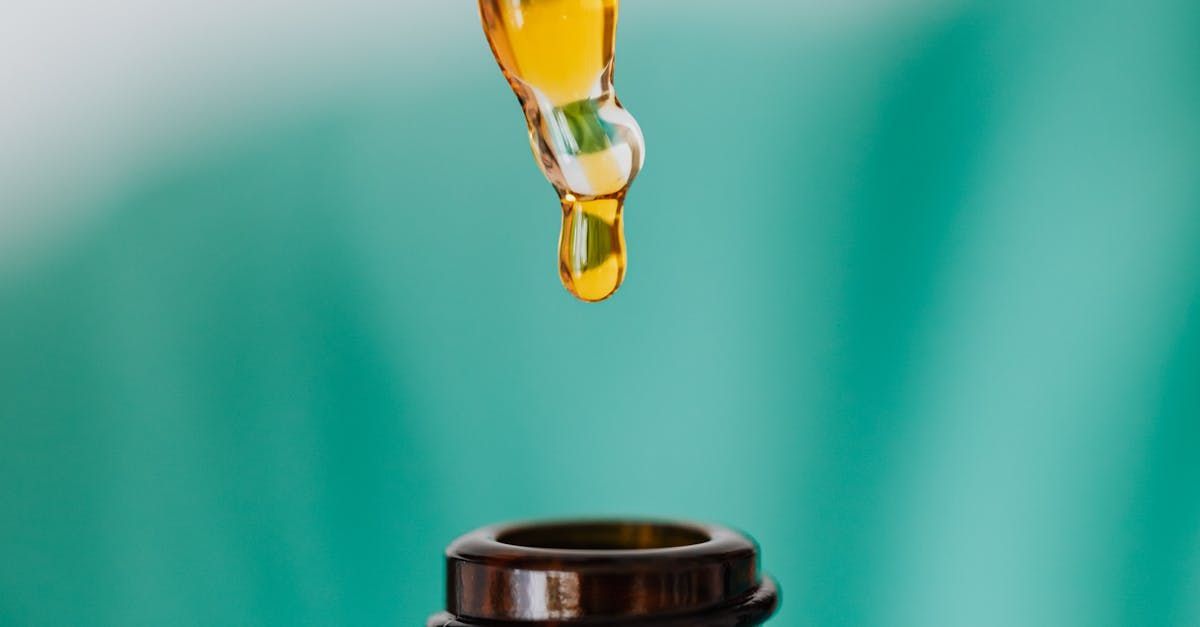
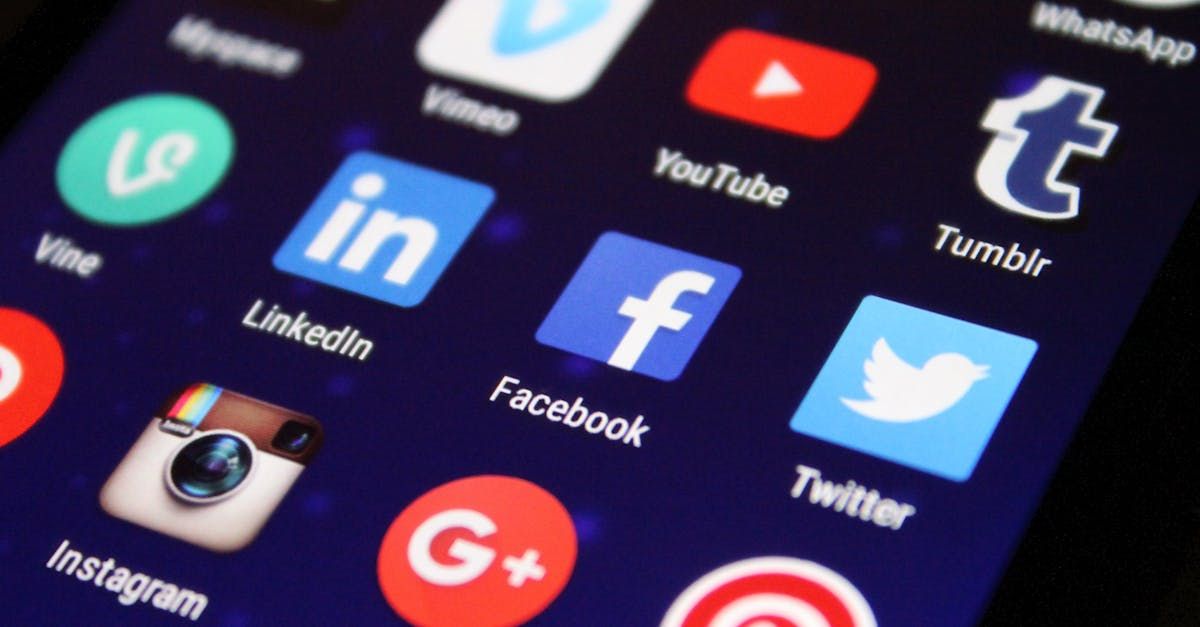